TIMS: A New Dimension in Protein Analysis
How machine learning is contributing to confident peptide identification in TIMS-based immunopeptidomics.
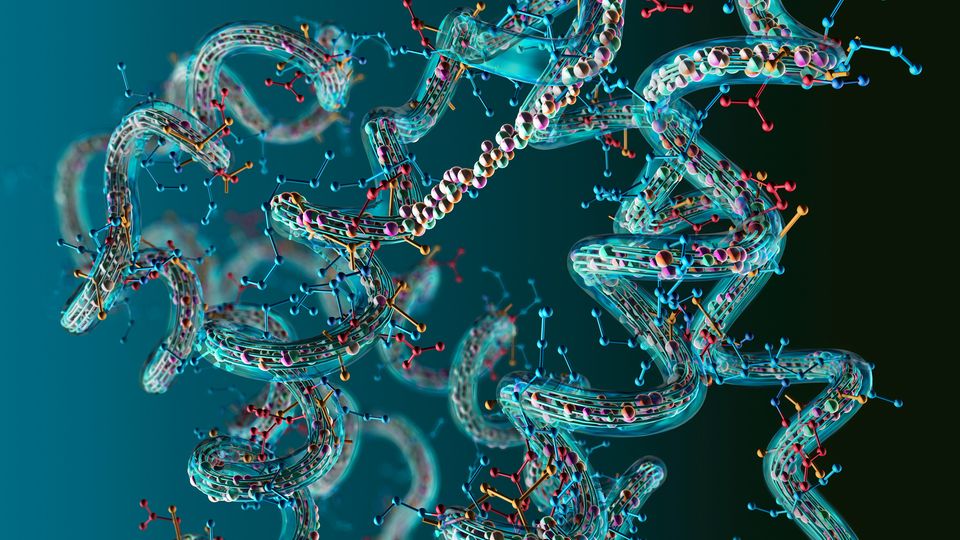
Complete the form below to unlock access to ALL audio articles.
This article includes research findings that are yet to be peer-reviewed. Results are therefore regarded as preliminary and should be interpreted as such. Find out about the role of the peer review process in research here. For further information, please contact the cited source.
Progress in analytical chemistry demands a symbiotic relationship: advances in analytical techniques are increasing the amount of data that we can collect, while developments in machine learning (ML) are streamlining the analysis and interpretation of those data. Trapped ion mobility spectrometry (TIMS), a recent evolution of traditional ion mobility spectrometry, is a notable example of a contemporary instrumental advance. In TIMS, an electric field is applied to trap and elute ions based on their mobility over time.1 The combination of TIMS with time-of-flight (TOF) mass spectrometry (MS) allows researchers to gather both mobility and mass data, which can be combined to leverage insights into the structure, function and interactions of proteins and other biological molecules. This approach is particularly successful for the analysis of proteins with similar structures and mass-to-charge (m/z) ratios, including those with post-translational modifications (PTMs) like phosphorylation.
TIMS-TOF adds a crucial additional dimension to protein analysis, allowing analysts to transition from 3D to 4D proteomics. The result is increased sensitivity, selectivity and acquisition speed. By establishing optimized analysis strategies, in particular the combination of TIMS-TOF and data-independent acquisition parallel accumulation-serial fragmentation (PASEF) (dia-PASEF), the additional information provided with this 4D approach is particularly useful for complex samples. This is partly due to the ability of TIMS to elute molecules in dense, separated clusters based on the presence of shared structural elements.
This article explores application areas that are showing the most potential for this technology and illustrates how ML approaches are being applied to maximize its value.
A collision course for success
TIMS separates ions based on collisional cross section (CCS) values that are intrinsic to each analyte’s structural properties and are highly reproducible across instruments and labs. As analytes are separated according to their CCS values, they are also separated in terms of certain structural properties, then analyzed via MS and identified. Large collections of such measured peptide sequences and their CCS values can be used by ML to generate generalized models for the prediction of CCS values, which contributes to the general understanding of ion mobility. For example, with proteins, hydrophobicity and the positions of histidine and proline residues are thought to be the main determinants of CCS, as well as sequence-specific interactions.2
A core challenge in protein analysis is the conversion of analytical data into tangible peptide spectrum matches (PSMs), through which analytes are identified as peptides by comparing them with existing databases. In the simplest scenario, database search algorithms use precursor and fragment ion spectra to identify a “best fit” match with an associated probability score. In many cases, the probability score will be similar for multiple matches, but only one peptide will be chosen. The result: false identifications. However, comparing the experimental CCS values obtained via TIMS to the ones predicted by ML models – thus punishing outliers and benefiting perfect matches with weak support – can be used to boost the number of PSMs, peptides and proteins identified in bottom-up proteomics applications, increasing confidence in the resulting assignments.
Application in immunopeptidomics
TIMS-TOF has been successfully applied in various ‘omics fields, such as proteomics (including at the single-cell level), lipidomics and metabolomics, and the technique may be particularly powerful when applied to immunopeptidomics. Immunopeptidomics refers to the analysis of peptides that when presented to T cells trigger an immune response to fight infection. Importantly, MS-based immunopeptidomics represents the only unbiased method available for the identification and characterization of these peptides3 and can reduce the number of cells needed from millions to just a few thousand. The CCS-enabled specificity of TIMS-TOF also ensures the precision of immunopeptidomic results.
One team has applied its TIMS-TOF system to the sensitive, high-throughput immunopeptidomic analysis of human leukocyte antigen (HLA)-associated peptides, setting the benchmark in the field.4 Personalized cancer vaccines and cell therapies targeting HLA peptides have demonstrated promise in early trials; thus identification of these peptides could inform the development of new cancer therapies. The researchers identified more than 15,000 distinct peptides using only one million cells, and without the need for fractionation. When applied to tumor-derived samples, the method enabled sensitive, high-throughput, and reproducible profiling of clinically relevant peptides from around 15 mg of wet weight tissue. The authors state that their method could be used to drive immunopeptidomics profiling in large patient cohorts and improve CCS prediction algorithms for HLA peptides.4
More recent work using TIMS-TOF has resulted in a new highly-sensitive, automated and economical workflow for HLA peptide analysis, termed immunopeptidomics by biotinylated antibodies and streptavidin (IMBAS).5 IMBAS-MS quantifies more than 5,000 HLA class I peptides from only 200 µL of plasma, in just 30 minutes. Using this approach, it was observed that the plasma immunopeptidome of healthy donors is remarkably stable throughout a year and strongly correlated between individuals with overlapping HLA types. Immunopeptides originating from diverse tissues, including the brain, are proportionately represented. Having established the basic workflow, the research team then looked at integrating the data into ML predictive models. Despite noting that more work was needed, they concluded that sHLAs offer a promising avenue for immunology and precision oncology.
In addition, in the world of de novo sequencing, where analysis, assembly and sequence prediction bioinformatics tools rely on ML/deep learning-driven algorithms, advances in TIMS-TOF instruments have allowed critical advances in the construction of larger training datasets. As in many ML applications, the quality and size of the training dataset is critical for subsequent data processing. Work reported in 2021, for example, indicated that increasing the training dataset from around 50,000 samples to just under 280,000 samples resulted in a decrease in relative error of sequence prediction of more than 20%.2
Machine learning for proteomics
ML already forms an integral part of many analytical workflows and has been applied to almost all stages of MS-based proteomics, contributing to the development of a truly multi-dimensional data landscape in this fast-developing field. Applications of ML in proteomics include multi-level data integration from databases, text and medical records, for example, and biomarker discovery from extensive datasets.6 The overall aim is the simplification of data analysis, improving efficiency and reducing the time needed to obtain results.
With good ML models now becoming established, workers are looking to advance performance further, building larger reference datasets, and exploring data analysis routines to improve the rate of identification of interesting peptides, for example.
One group, working in the field of immunopeptidomics, recently reported how, by improving rescoring – a powerful enhancement of standard sequence database searching – they were able to demonstrate up to 3-fold improvement in the identification of immunopeptides, even from low input samples.7 The research team trained a deep learning-based fragment ion intensity prediction model using more than 300,000 synthesized non-tryptic peptides from the ProteomeTools project. Samples were analyzed by TIMS-TOF to generate a dataset that was used to fine-tune an existing Prosit model.
Notably, in the case of data-independent acquisition (DIA), which focuses on quantification rather than identification, ML methods can reduce the m/z range in which researchers look for signals, increasing the quality of the data points analyzed.
Looking ahead
TIMS is a well-established ion mobility technique that facilitates selectivity, sensitivity and speed in complex analyses – including those of single cells and the immunopeptidome. Advances in this field have come rapidly, largely because of advances in ML-based approaches focused on outcome prediction and data handling. While researchers have been used to conducting both analyte identification and quantification studies, now, peptide values, including their CCS values, can be predicted based on their primary sequence to a degree of confidence almost as high as through direct measurement, facilitating potential quantification without prior identification.
Coupling ML approaches and increasingly innovative analytical instruments will allow researchers to gain previously unimaginable insight into the complexity of biological systems. This new level of understanding looks set to deliver clear benefits in the development of biomarkers and treatments for disease, and the next advances in ML may push our knowledge further beyond our current comprehension.
About the authors:
Jonathan Krieger is the head of research for Bruker ProteoScape, Bruker’s bioinformatic platform to analyze dia-PASEF and dda-PASEF timsTOF data in real-time. He has been at Bruker since November 2021 and has experience both in the biological and computational aspects of proteomics analysis.
George Rosenberger obtained his PhD in the group of Ruedi Aebersold at ETH Zurich, developing novel algorithms for the analysis of DIA/SWATH profiles. After a post-doc in computational systems biology at Columbia University, he held different academic and industry positions, focusing on innovative proteomic applications. At Bruker, he is working at the intersection of computational proteomics and machine learning.