Lab of the Future: Digitization, Automation and Lab Sustainability
eBook
Published: December 14, 2023
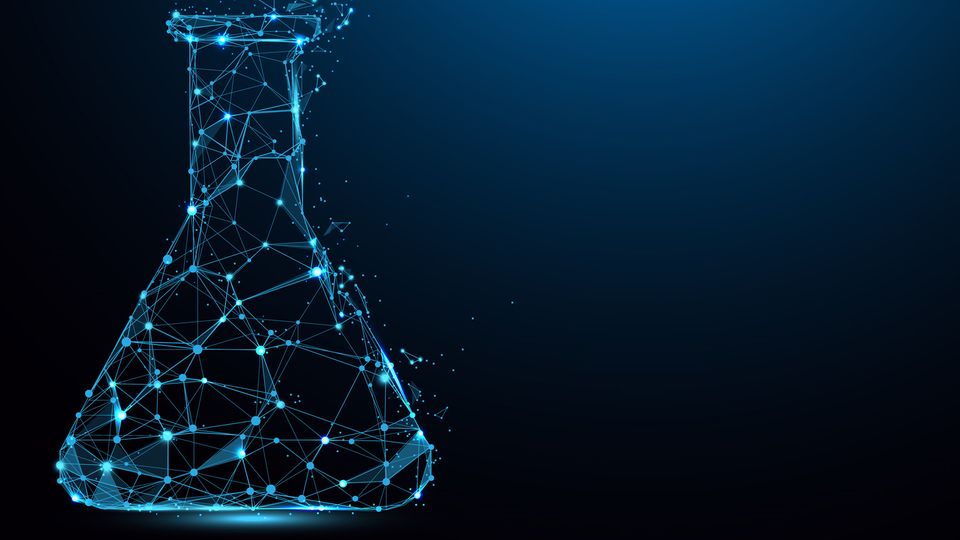
Credit: Technology Networks
Years from now, what will laboratories look like? Advances in digitization, automation, artificial intelligence and machine learning, along with the integration of sustainable practices, are changing how labs are built and run from the ground up.
This eBook explores the technologies that will feature and enable the next generation of laboratories and researchers.
Download this eBook to discover topics including:
- How To Future-Proof Your LIMS: Handling Software Updates
- New Advances in the Bioprocess Pipeline
- Lab Sustainability: A Holistic Approach
SPONSORED BY
Lab of the Future:
Digitization, Automation
and Lab Sustainability
Data Reporting: Connecting
the Islands of Automation
Towards the Lab of the
Future
How To Future-Proof Your
LIMS: Handling Software
Updates
Credit: iStock
Technologies Helping To Drive Digital Transformation
and Laboratory Efficiencies 4
How To Reduce Data Integrity Risk 8
Towards the Lab of the Future 13
New Advances in the Bioprocess Pipeline 16
Infographic: The High-Throughput Technologies
Speeding Up Biomedical Science 19
Data Reporting: Connecting the Islands of Automation 21
The Future of Digital Health With Professor Michael Snyder 25
Lab Sustainability: A Holistic Approach 27
Failing Faster, Succeeding Sooner: Technologies
That Are Accelerating Drug Discovery 30
How To Future-Proof Your LIMS: Handling Software Updates 35
Contents
2 TECHNOLOGYNETWORKS.COM
SYNTHIA™ Retrosynthesis Software is a unique web-based
platform that revolutionizes the way organic chemists
design pathways to complex targets. This decision-support
tool is the strategic partner of synthetic organic chemists.
Pioneer Your Pathway
Go Beyond
the literature
www.SynthiaOnline.com
MK_AD12813EN Vers. 01 09/2023
© 2023 Merck KGaA, Darmstadt, Germany and/or its affi liates. All Rights
Reserved. Merck, the vibrant M, Sigma-Aldrich and SYNTHIA are trademarks
of Merck KGaA, Darmstadt, Germany or its affi liates. All other trademarks
are the property of their respective owners. Detailed information on
trademarks is available via publicly accessible resources.
The Life Science
business of Merck
operates as
MilliporeSigma in the
U.S. and Canada.in
the U.S. and Canada.
4 TECHNOLOGYNETWORKS.COM
Technologies Helping To Drive
Digital Transformation and
Laboratory Efficiencies
Lee Baker
The digital transformation of laboratories is a rapidly
evolving field, with advances in automation and
digitization fundamentally changing the way labs are
designed, built and run from the ground up. Several
technologies are helping to drive this transformation,
ranging from artificial intelligence (AI) to laboratory
information management systems (LIMS).
Overall, the integration of these technologies is enabling
labs to operate more efficiently, with increased speed,
accuracy and flexibility. Labs will be able to leverage these
advances to accelerate the pace of scientific discovery and
drive innovation in their respective fields. In this article,
we highlight some of the key technologies fueling digital
transformation and explore the impacts they are having on
laboratory efficiencies.
Digitizing the laboratory
Modern lab managers are acutely aware of the benefits
that digitization can bring to their operations. Firstly,
digitization improves data management by providing
a centralized platform for storing, accessing and
analyzing data. This allows for efficient data sharing
and collaboration among researchers, facilitating faster
decision-making and accelerating scientific discovery.
Additionally, digitization enhances laboratory efficiencies
by automating manual tasks and streamlining workflows.
It reduces human errors, increases productivity and
frees up researchers’ time to focus on more complex and
critical tasks. Moreover, digitization enables real-time
monitoring and analysis, providing valuable insights
and enabling proactive decision-making. This leads to
improved quality control, optimized resource utilization
and reduced costs. Furthermore, digitization enhances
compliance and traceability by maintaining comprehensive
records and audit trails. It supports reproducibility and
data integrity. Overall, digitization offers laboratories the
potential to improve their research capabilities, enhance
collaboration, increase efficiency and accelerate scientific
progress. Advances in digital technologies such as LIMS
and electronic lab notebooks (ELNs) are already helping
to drive the digitization of laboratories and improve the
accuracy and reproducibility of scientific research.
Improving data quality with LIMS
LIMS are comprehensive software systems designed to
manage laboratory workflows, data and samples, offering
significant advantages to laboratories. One key benefit
of LIMS is their ability to streamline operations. LIMS
provide a centralized platform for managing all aspects of
laboratory activities, from sample collection and tracking
to data analysis and reporting. By automating routine
tasks such as sample registration, labeling and tracking,
LIMS eliminate the need for manual data entry and reduce
Lab of the Future
Credit: iStock
5 TECHNOLOGYNETWORKS.COM
Lab of the Future
Credit: iStock
the chances of human errors. This automation leads to
increased productivity as laboratory staff can focus on
more critical tasks and scientific analysis.
Furthermore, LIMS play a crucial role in improving
data quality within laboratories. By standardizing data
collection methods and enforcing data integrity rules,
LIMS ensure consistent and accurate data entry. Real-time
access to data allows researchers to retrieve information
quickly, facilitating decision-making and enabling timely
responses to experimental outcomes. Moreover, LIMS
offer features like data validation and data auditing,
enabling better quality control and compliance with
regulatory requirements.
The versatility of LIMS extends beyond data management.
They support workflow automation, ensuring that
laboratory processes follow predefined protocols
and reducing the potential for human error. With
customizable workflows, laboratories can define specific
steps and procedures, resulting in standardized and
efficient operations. LIMS can also aid in compliance
with regulatory guidelines and industry standards by
providing traceability and audit trails for samples, tests and
processes.
Additionally, LIMS generate comprehensive reports,
which are essential for documentation, analysis and
sharing results. Researchers can easily retrieve and compile
data for analysis, track experimental progress and generate
reports that meet specific requirements. This functionality
saves time and effort, streamlining the process of reporting
findings to stakeholders or regulatory bodies.
“Labs produce and gather huge volumes of data,” says Dr.
Becky Upton President of the Pistoia Alliance. “These
datasets have the potential to give researchers much deeper
insight into their research questions and to make new
connections, but in order to gain insights and answers
from this data, we need to make sure it is stored according
to best practice FAIR principles (findable, accessible,
interoperable and reusable) and make it more easily
accessible through semantic enrichment,” she adds.
LIMS – and especially cloud-based LIMS – can help labs
achieve this by providing a centralized repository for
data and by making it easy to search and analyze data.
Additionally, LIMS can help labs comply with regulations
by tracking and recording data in a secure and auditable
way.
Dr. Andrew Buchanan, principal scientist at AstraZeneca,
underscores that the keys in driving digital transformation
are “the ability to barcode and track individual molecules
from sequence, format, batch expression/QC and the
functional data that is generated with the asset. [These]
are essential for how we deliver the work flows we operate.
Without this, the error rate is high.”
Moving to the cloud
Cloud computing refers to the delivery of computing
services over the internet, enabling remote access to data,
software and resources. In the laboratory, cloud-based
LIMS can revolutionize data management and analysis.
By leveraging cloud computing, labs can store and analyze
large datasets, collaborate with colleagues and access
software and resources on-demand. Researchers can
conveniently access data and software from anywhere with
an internet connection, promoting remote collaboration
and enhancing flexibility. Furthermore, cloud computing
offers cost-saving advantages by reducing the need for
traditional IT infrastructure, such as servers and storage
devices. Instead of investing in expensive hardware and
software, labs can utilize cloud computing services and
pay for computing resources as needed. This cost-effective
approach allows labs to allocate resources efficiently
while leveraging the power and scalability of cloud-based
solutions.
For example, Carnegie Mellon University, in conjunction
with an industrial partner, recently built the world’s first
university cloud lab. In this endeavor, Carnegie Mellon’s
cloud lab is spearheading groundbreaking efforts to
harness the full potential of cloud computing. With a
specific focus on data management, the lab is developing
cutting-edge methodologies and tools that enable seamless
storage, retrieval and analysis of vast datasets. This
approach not only streamlines research processes but
also facilitates collaboration by empowering researchers
to access and collaborate on data from any location with
internet connectivity.
By leveraging cloud computing infrastructure, the lab is
optimizing resource allocation, enhancing computational
capabilities, and driving efficiencies across various
scientific and technological endeavors. Carnegie Mellon’s
researchers plan to use the digital space to pave the way for
advancements in fields ranging from AI and data analytics
to high-performance computing and Internet of Things
(IoT) applications.
Optimizing experimental design with AI
Over the years, AI has undergone significant evolution,
transforming the way scientists approach research and
experimentation. With its ability to learn from data,
recognize patterns and make informed decisions, AI has
become a valuable tool in scientific endeavors. It has the
potential to revolutionize various aspects of scientific
research and has already found applications in drug
discovery and diagnostics.
One area where AI excels is in optimizing experimental
design. By simulating different scenarios and predicting
outcomes, AI can assist researchers in identifying the most
6 TECHNOLOGYNETWORKS.COM
Lab of the Future
promising avenues of research. This helps in reducing
the time and cost associated with experimental design,
enabling scientists to focus their efforts on areas with
higher potential for success. For example, researchers at
the Broad Institute of MIT and Harvard are using AI to
design experiments to solve a gene therapy problem. The
Broad Institute’s AI screening technology, Fit4Function,
was 90% successful at finding viral vectors that don’t cause
disease but can deliver potentially life-changing gene
therapies to specific cells in the body.
In drug discovery, AI is being utilized to accelerate
the identification and development of new therapeutic
compounds. Machine learning (ML) algorithms can
analyze vast amounts of data, such as chemical structures
and biological properties, to identify patterns and predict
the efficacy of potential drug candidates. This enables
researchers to prioritize the most promising compounds
for further investigation, saving time and resources in the
drug development process.
Natural Language Processing (NLP) is a crucial
component of AI and ML. NLP enables machines to
understand and contextualize human language, opening
up new possibilities for scientific research. “In the future,
NLP-driven AI and ML will augment human researchers
in the lab, enabling them to uncover new relationships
between data and automate large analyses to accelerate
research and increase the success of drug development,”
explains Dr. Upton.
While automation tools and machine learning algorithms
are powerful, Dr. Buchanan notes that they are not
intelligent; “For me the intelligence comes from human
insight and the ability to ask the right question. Once
the question is agreed, then these tools come into play
and hopefully enable new more powerful insights and
discoveries.” By combining human expertise with AI
capabilities, scientists can leverage the full potential of AI
in advancing scientific knowledge and innovation.
Integrating technologies in the laboratory of
the future
The adoption of advanced technologies in the lab of
the future is not without its challenges. Labs often face
several barriers when considering the implementation
of these solutions. One significant barrier is the initial
cost associated with acquiring and implementing new
technologies. Advanced systems such as LIMS, robotics,
IoT devices and AI software may require substantial
investments in infrastructure, equipment and training.
Labs must carefully evaluate the costs and benefits
of adopting these technologies and ensure that they
align with their budget and long-term goals. Another
challenge is the compatibility and integration of new
technologies with existing systems and workflows. Labs
may already have established processes and legacy systems
in place, making it difficult to seamlessly integrate new
technologies. Ensuring compatibility, data interoperability
and a smooth transition from old to new systems requires
careful planning and coordination.
Additionally, labs must address concerns regarding
data privacy, security and regulatory compliance. As
technologies like cloud computing and IoT involve the
collection, storage and transmission of sensitive data, labs
must ensure robust cybersecurity measures and adhere
to regulatory requirements to protect the integrity and
confidentiality of their data. Furthermore, the adoption
of advanced technologies often requires a cultural shift
and changes in the mindset of lab personnel. Researchers
and staff may need to acquire new skills and adapt to new
ways of working. Training and education programs must
be implemented to familiarize lab members with the new
technologies and build their confidence in utilizing them
effectively. Collaboration and knowledge sharing among
labs can also be a challenge. Labs working in isolation
may struggle to access shared resources, collaborate on
projects, or exchange best practices. Overcoming these
barriers requires establishing networks, partnerships and
platforms that facilitate communication, collaboration and
the sharing of resources and knowledge.
Other technologies driving the lab of
the future
Robotics: Automated systems in the lab
perform repetitive tasks, improving precision
and freeing up researchers’ time. They
offer advantages like increased accuracy,
reproducibility and the ability to handle
hazardous materials or sterile conditions.
Internet of Things (IoT): IoT connects devices
and sensors, enabling real-time data collection
and analysis. It optimizes lab operations,
improves quality control, automates routine
tasks and provides critical insights into
experiments and processes through data on
environmental factors.
Virtual and Augmented Reality (VR/AR):
VR immerses users in simulated environments
using head-mounted displays and interactive
devices. AR overlays digital information
onto the real world through mobile devices
or smart glasses. Both VR and AR enhance
data visualization, accelerate discovery and
improve efficiency by providing immersive and
interactive experiences for researchers.
7 TECHNOLOGYNETWORKS.COM
Lab of the Future
Credit: iStock
Dr. Buchanan recommends having an expert team of
automation engineers and data scientists to implement
these technologies that “should integrate well with
experimental colleagues, scripting existing protocols,
suggesting modifications more suited to automation.
These protocols need to be established, validated and
maintained.”
It is interesting to note that the COVID-19 pandemic has
significantly impacted workflows in labs and accelerated
the adoption of digitization in scientific research. With
physical distancing measures and restricted access to
lab facilities, researchers had to find alternative ways to
continue their work and maintain productivity. This led
to a greater reliance on digital tools and technologies.
One key aspect of digitization that became crucial during
the pandemic was remote access to data and software.
Labs quickly embraced cloud-based solutions to enable
researchers to access their data and analysis tools remotely,
ensuring that research projects could continue even when
physical access to the lab was limited. Furthermore, labs
increasingly adopted LIMS to streamline data collection,
storage and analysis. Digital solutions provided centralized
repositories for data, improved data integrity and enabled
efficient collaboration across teams, even when physically
dispersed.
These experiences will likely have a lasting impact, with
labs continuing to leverage digital technologies to enhance
efficiency, collaboration and adaptability in the postpandemic era.
Dr. Upton highlights the impact of the pandemic on labs,
which accelerated the field of automation and robotics,
and notes that we can expect even more in the next 12
months; “The COVID-19 pandemic encouraged many labs
to explore the use of automation, robotics and VR and
AR. Huge benefits are to be reaped from standardizing
and connecting up laboratory data, making research more
efficient and less costly, while the rise in automated labs
that can be operated 24 hours a day will ultimately lead
to significant productivity gains. Over the next 12 months
we expect to see even greater levels of automation and
labs emerging that are completely driven by AI-powered
robotics.” OpenAI’s ChatGPT could be a game-changer,
says Upton, but “with any new technology, from the outset
it’s important to take the time to understand the potential
risks and implications of its use.”
Transforming the laboratory for the better
LIMS, robotics, IoT, AI, cloud computing and VR/AR
are powerful tools for labs looking to optimize their
operations, improve their data quality and streamline their
workflows, making them critical technologies driving
digital transformation in laboratories.
By optimizing experimental design, providing real-time
data and analytics, automating routine tasks, reducing
errors and freeing up researchers’ time, these technologies
can significantly improve laboratory efficiencies, ultimately
accelerating scientific discovery and innovation.
On the lab of the future, Dr. Upton insists that “technology
has the potential to transform the laboratory for the better,
but only if our industry is prepared to invest in the people
and processes that must accompany it.”
8 TECHNOLOGYNETWORKS.COM
How To Reduce Data Integrity Risk
Bob McDowall, PhD
Data integrity problems continue to plague the
pharmaceutical industry – especially in regulated
laboratories – with violations still being identified by
health authority inspectors over 18 years after the Able
Laboratories fraud case.1
This is despite guidance issued
by various regulators2,3,4,5,6
and industry bodies7,8,9,10,11
that include advice for ensuring that data and records are
protected and comply with applicable regulations.
The aim of this guide is to provide practical advice on how
to reduce data integrity risk and ensure GxP compliance.
We will learn from those in the industry who are expert
at failing to comply with data integrity regulations and
who have been on the receiving end of an FDA inspection.
We will use citations from a recent and extensive 483
observation issued to a single organization in December
2022.12
We will present and analyze selected citations to identify
the reasons for failure. We will then suggest ways to
prevent reoccurrence or, for readers who are more
proactive, prevent the issue from arising in their own
laboratories. Some remediation suggestions will involve
laboratory automation. Before senior management has
collective heart failure in response to this proposal, know
that automation will be accompanied by improvements
in business benefits that will more than offset the cost,
including validation.
The approaches outlined here could also be useful for nonregulated laboratories to improve efficiency.
Manual colony counting
Observation 1 item 2 in the citation targeted the practice
of manual colony counting on microbiological plates. This
process is slow and error prone and inevitably generates
regulatory interest. The relevant section of the citation
reads:
Laboratory records do not include complete data derived
from all tests … to assure compliance with established
specifications and standards. For example,
1. Environmental monitoring samples were not counted
accurately. On November 22, 2022, review of plates
from QC1 that had been counted by one analyst and
checked by a second analyst found the reported result
to be less that the number of colonies on the plate.
2. Microbiology personnel reported the laboratory
practice was to count colonies that merge together,
with similar morphology, as one colony. Review of
plates showed this practice resulted in under counting of
colonies.
12
Under reporting of colonies means that potential out of
specification (OOS) results are hidden and failing batches
are passed or rooms that are meant to be clean become
potential sources for microbiological contamination. After
manual counting and a second-person review, the plates
are thrown away after with no record other than what is
written down in the analytical batch record. This provides
no objective record for an inspector or an auditor to assess
if the recorded value is correct. The inspectors were able to
review current plate counting on a specific day before the
plates were discarded.
Lab of the Future
Credit: iStock
9 TECHNOLOGYNETWORKS.COM
Lab of the Future
Credit: iStock
What the citation does not state is by how much the counts
were under reported or if it was deliberate. However,
counting merged colonies is unscientific and deliberate
falsification.
A solution is to use an automated colony counter that
also produces a photographic record for each plate
counted. Simple manually fed colony counters can read
and photograph one plate at a time. Depending on the
number of plates to be read, an automated plate feeder in
combination with a bar code reader to positively identify
plates would be a better option as it removes labor from the
process. Instrument purchase and validation costs will be
offset with the gains in productivity.
Lack of instrument printouts
An apocryphal good manufacturing practice (GMP) saying
is that if it’s not written, it’s a rumor, which can be modified
for this example, to if it’s not printed, it’s a rumor, as seen in
the next 483 citation from observation 1 item 3:
b) pH printouts and recording of description associated
with the <redacted> and <redacted> samples from
<redacted> could not be provided.
12
This is gross incompetence indicating a lack of basic GMP
training and a total failure of the pharmaceutical quality
system. Systematic failures here were:
1. The analyst failed to document their work and failed to
print the pH values obtained for an instrument check
and sample analysis.
2. A failure of design in the analytical batch record,
which should have prompted the analyst to document
these results.
3. The second-person reviewer failed to identify the lack
of documented evidence.
4. Training of the performer and reviewer has failed
totally.
5. Procedures were not followed or not created in the
first place.
6. Quality oversight is conspicuous by its absence.
If this is the situation for pH measurement, what other data
chasms exist in this laboratory, especially for more complex
analyses? I’m not convinced that retraining analysts and
reviewers would work and therefore automation is a prerequisite to solve the problem.
Long-term remediation requires an informatics solution
to automate and enforce working practices and ensure
that data are collected from any interfaced instruments.
The analyst would not be able to proceed with the analysis
unless the instrument measurement was captured by the
application. A solution should not require an analyst to
press a SEND button on the instrument, as this allows
them to select a “correct” result and all that is gained is
electronic data falsification. Automate the whole process.
Paper records would also be eliminated by taking this
approach, which would speed the analysis and review but
also enable verifiable quality oversight.
Uncontrolled manual chromatographic peak
integration
In chromatography, peak integration is a critical process
requiring control of manual integration parameters due to
multiple instances of falsification found during inspections
since 2005.1
This is an area of great regulatory interest.
Inspection of chromatograms and peak integration
revealed inconsistencies that led to observation 2, item 1:
There is no procedure describing the use of manually
entered integration events, including baseline points,
tailing sensitivity and peak slice for processing
chromatography data … The reviewers only review the
final chromatogram and do not review the processed
chromatogram to ensure that the manually entered
integration events are justified.
Procedure SE/BQC/00165 Interpretation of
Chromatograms requires manual integration be
documented clearly stating the reason the manual
integration was performed and the initials of the section
head for approval. But when analysts manually enter
integration events to force the software to integrate in a
specific way, there is no similar documented justification
and approval process ... For example (this is one
of several observations that has been selected for
discussion here):
... Additionally, the 6-month accelerated time point
for the same lot <redacted> was integrated manually
by adding a fronting sensitivity and a tailing sensitivity
factor to the peak for impurity <redacted> but not
for the standard of the same impurity. This reduced
the area of the impurity compared and gave a result
of <redacted>% compared to a limit of <redacted>%.
When the fronting and tailing sensitivity factors are
removed to ensure integration of the impurity compared
with the standard, the reportable result changes to
<redacted>%, a value that would have required an
investigation.
12
First and foremost, there needs to be a procedure for
integration of chromatographic peaks that is applicable
to all laboratory staff. Changes made by an analyst must
be justified. The journey from first to last processing must
be traceable and complete to ensure data integrity. While
there is a procedure, it was not followed.
The major problem with the laboratory work is a
failure to be scientifically sound as required by 21 CFR
10 TECHNOLOGYNETWORKS.COM
Lab of the Future
211.160(b): … the establishment of scientifically sound
and appropriate specifications, standards, sampling plans,
and test procedures …13 Chromatography is a comparative
and not an absolute analytical technique, therefore
standards and samples must be integrated the same way.
As the inspectors found, treating samples and standards
differently has hidden an out-of-specification result and
avoided an investigation. This is data falsification.
We have moved on from test injections in the early days of
data integrity violations to subtle changes in integration
that may be difficult for an auditor or inspector to identify
unless they take sufficient time to look in detail at manual
peak integration.
Resolution may be found by using technical controls
to limit manual integration for main assays within a
chromatography data system. However, there is a problem
with impurity analysis as we may be very close to limits
of quantification and manual integration maybe the only
way of measuring peaks. Training is essential along with
effective review and quality oversight, coupled with
management leadership about what are acceptable and
unacceptable practices. However, there is much doubt if
this company can achieve this.
Instrument logbooks are critical for data
integrity
Instrument maintenance and use logbooks are mandatory
under both US and EU GMP regulations13,14 and are
the unsung heroes of data integrity. Logbooks provide
corroborating evidence of activities performed with
instrumentation and are also used to correlate activities in
the controlling computerized system including the audit
trail entries with printouts from the system. If the system is
electronic or there are only printouts from an instrument,
the role of the logbook remains the same.
The 483 observation 3 item 1(a) is quoted at some length for
you to understand that instrument logs must be completed
and analysts must be trained to do this every time with
no exceptions. Reviewers also must be trained to ensure
that entries are complete and consistent. As for QA and
Management they are conspicuous by their absence except
when it comes to making excuses.
There were no weight-specific entries in your LIMS
Instrument Usage Logbook for the time recorded in
any of the balance printouts. Your Associate Executive
Vice-President of Corporate Quality and Compliance
and Manager of QC stated that the weighing activities
recorded on the Instrument Usage Log is not a true
representation of samples weight with start and end time
for each weighing activities for a specific lot of a product.
Further, there is no consistency among QC employees
in term of recording information in LIMS logbook
pertaining to a total number of lots tested. Some QC
employees may enter this information whereas others
may not, leaving no traceability for the exact number of
lots tested and their start and end time of analysis.
This issue is applicable to all analytical instrument in your
QC laboratory … For example, the time stamp on each of
the balance printout and <redacted> spectrum did not
match with your LIMS Instrument Usage Log record for
samples weighed and analyzed. There follows a list of time
differences, torn balance printouts, suspicious balance
weights between “real” and torn printouts. 12
To have an associate executive vice-president of corporate
quality and compliance state to an FDA inspector that
only some analysts complete logbook entries implies that
the individual knows of the problem. The individual is in a
senior management role with the power to do something
but has done nothing about it.
The main problem is that instrument logbooks are still
paper and must be completed manually. A suggested fix
involves incorporating automatic instrument logs, like
audit trails, into instrument data systems but software
suppliers only respond to market forces.
Have you got a GMP shredder?
I leave the worst citation to the last as Observation 3, item 1
has the following citation:
The responsibilities and procedures applicable to the
quality control unit are not fully followed.
As we shall see this is a vast understatement.
Specifically, there is a cascade of failure in your Quality
Unit’s lack of oversight on the control and management
of GMP documents that are critical in ensuring the drug
products manufactured and tested at your site are safe
and effective.
Summarizing the observation: QC, Production and
Engineering employees destroying GMP documents
by tearing it into pieces and disposed in scrap areas.
Additionally, we found a truck full of transparent plastic
bags containing shredded documents and black plastic
bags containing document torn randomly into pieces…12
This is industrial-scale destruction and falsification
of records for which there are no excuses. The 483
observation identifies several torn records in detail as well
as attempts to hide destroyed evidence and lying to the
inspectors.
My resolution for this violation is very simple. Fire all
employees and raze the facilities to the ground.
11 TECHNOLOGYNETWORKS.COM
Lab of the Future
Summary
There is an apocryphal phrase never assume malice
when stupidity will suffice. However, the citations in this
483 Form provide ample evidence of both malice and
stupidity.12 This conclusion arises from evaluating the
systematic problems with the pharmaceutical quality
system, lack of record keeping, inconsistent documents,
inadequate review in the lab coupled with abysmal
quality and management oversight highlighted in the 483
observations. Many of the observations discussed suggest
incompetence, and we have highlighted how automation
can be used to remediate these processes.
However, peak integration manipulation, manual colony
counting, hiding destroyed records, lying to inspectors
and the evidence of a truck full of shredded and torn GMP
records being driven off-site shows that malice in the form
of industrial scale falsification is abundantly present.
Learn from this company to avoid data integrity citations.
References
1. United States Food and Drug Administration. Able
Laboratories Form 483 Observations. 2005.
2. Medicines and Healthcare products Regulatory Agency.
MHRA GMP Data Integrity Definitions and Guidance for
Industry 2nd Edition. 2015.
3. Medicines and Healthcare products Regulatory Agency.
MHRA GXP Data Integrity Guidance and Definitions. 2018.
4. World Health Organization. WHO Technical Report Series
No.996 Annex 5 Guidance on Good Data and Records
Management Practices. 2016.
5. United States Food and Drug Administration. FDA Guidance
for Industry Data Integrity and Compliance With Drug CGMP
Questions and Answers. 2018.
6. Pharmaceutical Inspection Convention / Pharmaceutical
Inspection Cooperation Scheme. PIC/S PI-041 Good
Practices for Data Management and Integrity in Regulated
GMP / GDP Environments Draft. 2021.
7. International Society for Pharmaceutical Engineering.
GAMP Guide Records and Data integrity. 2017.
8. International Society for Pharmaceutical Engineering.
GAMP Good Practice Guide: Data Integrity - Key Concepts.
2018.
9. International Society for Pharmaceutical Engineering.
GAMP Good Practice Guide: Data Integrity by Design. 2020.
10. Parenteral Drug Association (PDA). Technical Report 80:
Data Integrity Management System for Pharmaceutical
Laboratories. 2018.
11. Active Pharmaceutical Ingredients Committee. Practical
risk-based guide for managing data integrity, version 2 2022.
12. United States Food and Drug Administration. Intas
Pharmaceuticals Limited Form 483 Observations. 2022.
13. United States Food and Drug Administration. 21 CFR
211 - Current Good Manufacturing Practice for Finished
Pharmaceuticals. 1978.
14. European Commission. EudraLex - Volume 4 Good
Manufacturing Practice (GMP) Guidelines, Chapter 4
Documentation. 2011.
Did you know you can access details about your instruments on service contracts from your PC or mobile device?
It's all about the data!
Gain transparency and visibility into all
aspects of your instruments enabling
you to make faster and more informed
business decisions to improve your lab.
With PerkinElmer's OneSource Portal web
and mobile app you can view all of the data
relating to your instruments such as serial
numbers, year of manufacture, past and
scheduled service events, and contract status
all through an easy to use interface.
Exclusive access to the mobile app is included with your Portal subscription.
Additional features included with the mobile application:
• Use voice and text capabilities to
describe issues well as upload images
to describe service issues
Some additional capabilities in the OneSource Portal are:
• Quickly generate a service request
• Share service details with colleagues
• Receive notifications about upcoming
instrument events such as PM and
service activities
Improve your lab operations with the OneSource® Portal and Mobile App
STAY CONNECTED
TO YOUR LAB
Laboratory Services
PORTAL
Web-based access for all of
your OneSource needs
MOBILE APP
Mobile access
to your instrument
repair status
To learn more please contact your local PerkinElmer representative.
For a complete listing of our global offices, visit www.perkinelmer.com
Copyright ©2023, PerkinElmer, LLC. All rights reserved. PerkinElmer® is a registered trademark of PerkinElmer, LCC. All other trademarks are the property of their respective owners.
96600
PerkinElmer, LLC.
www.perkinelmer.com
• Scan instrument service tags to
quickly open service requests
• View upcoming service events
• Create instrument 'favorites'
• View service history and and generate
reports for individual instruments or
your entire fleet
13 TECHNOLOGYNETWORKS.COM
Towards the Lab of the Future
Joanna Owens, PhD
In the lab of the future, researchers will be freed from
manual, repetitive experimental tasks, as automated
tools and artificial intelligence-powered robots carry out
protocols, collect and analyze data and design subsequent
experiments, freeing up time for humans to focus on
interpreting what the results mean and addressing the
bigger scientific questions.
The lab of the future will bring together a range of different
technologies, all digitally connected and seamlessly
integrated. These innovations will be involved in every step
of the research cycle, from managing a lab’s supply chain
of scientific products and reagents – handling samples,
chemicals and equipment – to sharing data within and
across organizations.
But the timescale for realizing this vision is slower in
some research sectors than others. In this article, we look
at the barriers preventing more widespread adoption of
automation and digitization, and the opportunities they
could bring.
Automating the academic lab
Anyone who has worked in a lab will be familiar with
the repetitive, manual nature of many experiments, and
there seems ripe opportunity for using automation to
free up researchers’ time. But for academic labs, adopting
automation can be daunting and cost-prohibitive, and isn’t
helped by structures for funding and impact assessment.
“I think the vision of the lab of the future differs in
academia and industry because we have different
outputs,” says Dr. Ian Holland, an engineer who moved
from the automation industry to a lab focused on tissue
biofabrication at the University of Edinburgh and
has written about the “automation gap” in academia.
“Academic labs tend to carry out a wider range of work and
there’s considerable protocol variability, whereas industry
uses standardized protocols for highly focused, repetitive
applications, which are more amenable to automation.
Academic labs cannot afford to invest in off-the-shelf
technologies that aren’t more flexible to suit their needs.
So, although there is appetite for the improved efficiency
that automation brings, the route to the lab of the future for
academic labs is less clear.”
There is a shared vision though, which is a world where
scientists spend more time doing science and automation
carries out the manual tasks. “It’s not good having highly
educated people carrying out manual tasks, and I think
that happens too much in academia. I’d like to see more
manual tests done by machines and let scientists do more
science,” says Holland.
Barriers to adopting automation
The short-term nature of academic research funding does
not lend itself to investing in large-scale technologies
for modernizing the lab, says Holland, and although
investment in major infrastructure such as robotics will
improve efficiency, it is difficult to directly relate that to an
increase in the output of research papers – the main metric
used to measure a lab’s success – making the investment
hard to justify.
This is a problem also experienced by Prof. Ross King, at
Cambridge University, who has been working for several
Lab of the Future
Credit: iStock
14 TECHNOLOGYNETWORKS.COM
Lab of the Future
decades on ‘robot scientists’ – semi- or fully autonomous
robots that automate simple forms of scientific research,
from setting new hypotheses to automatically designing
and running efficient experiments to discriminate between
them. This futuristic type of research seems to divide funding
panels, who have tended to take a conservative view, and
existing university structures don’t lend themselves to the
collaborative, interdisciplinary nature of the work required.
“I think it’s slowly changing, and we’re getting traction in
different areas, especially now these ideas are being taken up
by the pharmaceutical industry,” says King.
Another challenge for academic scientists is a skills
gap, because automation and robotics requires an
understanding of mathematical models, machine learning
and engineering – expertise not every lab has easy access
to. And although automation brings efficiency, it also
brings with it new challenges, such as how to manage large
amounts of data.
This is where having the right expertise can help, as Prof.
Ola Spjuth, from Uppsala University in Sweden, explains:
“We have a big focus on trying to automate our entire
cell-based screening and profiling methods in the lab,
and this generates a lot of images. This scale of data can
scare a lot of researchers, but we have a background in
managing big data and using high-performance computing
clusters here, so we see large amounts of data as valuable.
We’re also not the typical life scientists in that we take an
engineering approach and have a multidisciplinary group
with experimentalists, data scientists and engineers.”
Using automation and AI to improve efficiency
and reproducibility
Spjuth took what he calls an unconventional strategy to
automating the lab in that they did not go out and procure
entire robotic installations from vendors, instead choosing
to buy individual equipment components and build the
system themselves using an open-source approach. “It’s a
lot more challenging than buying something off the shelf,
but we have full control of all steps in the protocol, and we
wanted a research environment that we can grow with and
update.”
So far, the main efficiency gains have not been the
envisioned capacity increase from using robots able to
work 24/7. “We are getting there,” says Spjuth, “but our
system still needs a lot of human support, and steps such
as cell culture are too expensive for an academic lab to
fully automate right now.” The major gain, he says, is in
reproducibility – every experiment is carried out in exactly
the same way.
In fact, alongside efficiency, reproducibility appears to be
one of the main drivers for automating research processes.
One of the goals of King’s work on robot scientists is to
improve the scientific method. “Machines in some ways
already do better quality science than humans because
what they do is recorded, explicit and clear,” says Ross.
“Human beings are often unintentionally sloppy about
what they do in experiments, and there’s a huge problem
with scientific reproducibility because experiments are so
susceptible to human error. Just like games on computers
have improved over the years, we think that in science, the
machines will keep progressing. Ultimately, they’ll be as
good as humans at science, and maybe even better.”
King has already developed two prototype robot scientists,
Adam and Eve. Adam was designed to carry out functional
genomics in yeast, assigning functions to the genome that
was sequenced back in 1996. Eve specializes in early-stage
drug design, using artificial intelligence to find compounds
to treat specific diseases.
“The way compound screening used to be done in industry
was you would make an automated assay to tell you if a
compound was likely to be good or not, and then you’d
screen a large compound library – maybe one million
compounds – and find a small number of hits to take
forwards. Then you’d start again with another assay and
library,” explains King. “But actually, that’s a missed
opportunity, because you’ve learned something during the
screen and you could use that insight to decide what to do
next.” By using quantitative structure-activity relationship
(QSAR) models and accumulating biological knowledge,
Eve was trained to find hits using only a small fraction of
the compounds in a library – speeding up the process and
make it more cost effective.
Now, King is working on the next iteration of the robot
scientist – called Genesis – as part of the Nobel–Turing
AI scientist grand challenge. The challenge is to develop
AI systems capable of making Nobel-quality scientific
discoveries autonomously at a level comparable, and
possibly superior, to the best human scientists by 2050.
Genesis is a scaled-up robot scientist with thousands of
micro-chemostats – tiny bioreactors where nutrients are
continually added to cells and metabolic end products are
continually removed. These will enable Genesis to run
more sophisticated experiments in parallel. “We need an
AI system to plan so many experiments and especially
hypothesis-led experiments, rather than just altering a
component and seeing what happens,” says King. “Here,
the robot is saying ‘I think change Y will do X to this
model, and then it conducts the experiment to see if the
hypothesis is true.”
Moving towards a digitized laboratory
In addition to adopting robotic solutions to improve
efficiency and reproducibility in the lab of the future,
many researchers are moving towards digitizing their
15 TECHNOLOGYNETWORKS.COM
Lab of the Future
labs, switching from paper-based systems to informatics
solutions such as laboratory information management
systems (LIMS) and electronic notebooks (ELNs). LIMS
enable researchers to keep track of data associated with
samples, experiments and instruments efficiently, as well
as actively manage lab processes, while ELNs digitize note
taking and can automate the data review process. Guidance
on good records and data management practices from the
World Health Organization (WHO) recommends that
hybrid systems – a combination of manual and electronic
systems – should be replaced by fully digitized systems at
the earliest opportunity.
Adopting informatics solutions such as LIMS can
offer laboratories several benefits, including helping to
improve performance, maximizing quality and ensuring
compliance requirements and regulations are met. They
can also remove repetitive, laborious steps in workflows
and reduce human error. The time savings can empower
scientists, allowing them to focus on more complex and
meaningful work.
Despite the benefits offered, barriers to adopting these
solutions and digitizing a laboratory remain. The cost of
subscriptions, new equipment and software, as well as
time to implement the solutions, can be prohibitive for
many laboratories, particularly in academia. “Accessibility
is also a huge barrier. Many academic laboratories aren’t
set up for the digital capture of laboratory information,
both from a hardware and software perspective,” Dr.
Samantha Pearman-Kanza, senior enterprise fellow at
the University of Southampton, told Technology Networks
previously. Problems with outdated equipment and
software compatibility can further limit the adoption of
digital technologies. In addition, “The lab can often be a
hostile place for technology,” said Pearman-Kanza. Space
for using laptops or tablets may be limited, and researchers
may be concerned about spills and accidents occurring.
Even things such as removing gloves to type notes rather
than jotting them in a notebook can be seen as prohibitive.
However, the continuing advancement of technologies
is likely to reduce these barriers and encourage greater
adoption of digital solutions in the lab of the future.
“Much like smart homes have become commonplace
in today’s society, so will smart labs. Users will be able
to control their laboratories by voice using smart lab
assistants, all of the laboratory systems will be seamlessly
linked together and users will have multiple options to
record their data via voice, tablets, phones or computers if
they wish,” envisaged Pearman-Kanza.
Taking small steps towards the lab of the
future
It might be another two decades before fully autonomous
robots are designing and conducting experiments in the
lab, but it’s never too early for academic labs to start their
journey towards automation, says Holland. “As an engineer
in a biology lab you can see the potential opportunities to
use technology to improve processes. However, I think too
often in academia, researchers strive for a magic machine
that does everything. But that is never how you develop
automation as an engineer, you build prototypes that carry
out each part of the process.”
Holland advocates starting small, by automating
something simple such as fluid dispensing that can bring
substantial gains in efficiency and reproducibility. In the
tissue biofabrication lab, just making this change has
reduced a protocol from 25 to 5 minutes, freeing up time
for other tasks.
Another advantage of adopting automation early is it can
help researchers looking to translate discoveries from
bench to bedside. “The earlier you can include automation
in your process and start thinking about that, the better
chance you have of convincing people to invest in your
product, because they can see it will be easy to scale up
quickly.”
In Spjuth’s lab they are hoping for additional collaboration
with other researchers working on their own robotic
and automated solutions for the lab, sharing protocols
and code. “With major advances in technology such as
3D printing and people now sharing code for these and
other applications, it is becoming possible for researchers
to do much more independently. The do-it-yourself
movement is advancing and that means you can build your
own microfluidic chips and microscopes, and as prices
for robots come down there is an opportunity for many
biological labs to adopt some sort of lab automation.”
However, an important consideration as this movement
advances, notes Holland, is sustainability. “There is already
a real problem with automated processes generating high
amounts of waste – a machine generating millions of
waste pipette tips, for example. I think this needs to be
considered more and certainly in the design stage, both
from an environmental perspective and to ensure supply
chains can meet demand.”
16 TECHNOLOGYNETWORKS.COM
New Advances in the Bioprocess
Pipeline
Aron Gyorgypal, PhD
The bioprocess landscape is an ever-advancing industry
looking to optimize the manufacturing of biologics. Of
key interest is addressing the challenges of high costs,
time-consuming assays and processing complexities.
These challenges are tackled with technological innovation
meant to streamline and optimize the production pipeline.
Here we delve into groundbreaking advancements
throughout the bioprocess pipeline that are poised to
advance bioprocessing.
High-throughput upstream process screening
technology
Early biologic production is known to come with high costs
as it takes time to evaluate an optimized process, including
the medium, feed, reactor conditions and purification
methodologies. A critical need is to speed up analytical
times while scaling down cell culture systems, to help
better assess cell lines for key attributes, while decreasing
the costs of goods manufactured. This will ultimately lead
Lab of the Future
Credit: iStock, Gyorgypal, A. doi: 10.7282/t3-1c02-k359.
Bioprocess scheme showing the
overall bioprocess scheme of
the upstream and downstream
unit operations, including early
clone clean selection and the
use of model and control along
the product pipeline.
17 TECHNOLOGYNETWORKS.COM
Lab of the Future
Credit: iStock
to accelerated production of innovators and biosimilars.
Both liquid handlers and microfluidics coupled with
analytical equipment such as ultra-high-performance liquid
chromatography (HPLC) and mass spectrometry (MS) have
been showcased as effective for achieving this goal.
A successful cell line has high productivity. Cell clones
that produce high titers allow for the production of
drugs in a higher quantity but in less time. While new
technologies enable the engineering of high titer
cell clones, there is still a need to screen these clones
quickly.1
The current paradigm for titer analysis depends
on chromatographic approaches which, even with the
newest ultra-high-performance liquid chromatography
(UHPLC) models, will take minutes to analyze one cell
line. However, a recent innovation by D’Amico et al. used
microfluidics coupled with electrospray ionization MS to
improve analysis time tremendously from 3 minutes to 25
seconds per sample.2
This is achieved using a multiplate
Protein A-based cleaning coupled with microdroplet
entrainment and subsequent analysis by native MS. The
authors also imply that this technology can measure key
product quality attributes. Unsurprisingly, microfluidics
has recently gained prevalence as a technology to aid in
high throughput automation with both MS and HPLC
techniques, as an avenue towards process automation and
away from the need of manual in-process testing.3
A common product attribute that needs to be monitored in
biopharmaceutical manufacturing is protein aggregation,
which is known to influence a product’s safety and
efficacy. During early-stage development, identifying
aggregation in scale-down cell culture systems can be
tedious, as the small sample volumes make aggregate
detection challenging. Scientists at the University of
Sheffield looked to overcome this hurdle by developing
an automated small-scale analytical platform workflow,
combining purification and aggregation analysis of protein
biopharmaceuticals expressed in 96 deep well plate
cell cultures.4Their workflow utilized a liquid handler
equipped with a protein-A PhyTip column for purification
and a size-exclusion chromatography (SEC) column for
aggregate monitoring. The data was then analyzed, and
cultures were ranked by their aggregation levels. This
high-throughput method, allowing up to 384 clones to be
screened in 32 hours, enables high aggregation phenotypes
to be eliminated from clone selection early on in cell line
screening and can be used for both monoclonal as well
as bispecific antibodies alike. Technology such as this
one showcases the utility of liquid handlers for highthroughput automated screening.
Downstream purification – New ligands for
multimodal chromatography
Downstream product processing accounts for over half
of the production cost for monoclonal antibodies, and
significant research is devoted to developing compact and
affordable purification processes. Scientists are looking
to innovate new chromatographic technologies using
novel ligands capable of improved separation for higherthroughput operation. Within separation science, attention
is put on multimodal ligands that combine multiple
techniques, such as hydrophobic, hydrogen binding and
electrostatic integrations, that work orthogonally or
complementary to affinity or conventional hydrophobic
and ion exchange chromatography.5
The use of multimodal
chromatography effectively removes process-relative
impurities, such as host cell protein (HCP), DNA,
aggregates and protein fragments.
Scientists at the North Carolina State University developed
a multimodal salt tolerant cation exchange (CEX)
membrane, which is the first of its kind based on nonwoven
fabrics to be published and used for product capture and
polishing of biologics.6
This membrane was produced
by coupling 2-mercaptopyridine-3-carboxylic acid
(MPCA) to a polybutylene terephthalate (PBT) nonwoven
fabric, modified by UV grafting of glycidyl methacrylate
(polyGMA). The membrane achieved higher biologic
binding and capacity versus commercial CEX multimodal
resins and may be an effective alternative to current
commercial resin columns. Future efforts by the team look
to expand on the success of this technology to bring more
purification applications, such as a low-cost Protein-A
alternative and peptide-based resins for HCP depletion.
Ultimately, technology such as this looks to disrupt the
current downstream process purification paradigm
to allow for low-cost, high-throughput purification of
biologics.
Establishing Multi-Attribute Monitoring
The complexity of biologics processing comes from the
multitude of heterogenous post-translational modifications
that can alter protein-based therapeutic efficacy and
safety. Using multi-attribute monitoring (MAM), a liquid
chromatography-mass spectrometry (LC-MS) based
method, allows for direct characterization and monitoring
of numerous product quality attributes at the amino acid
level. Simultaneously monitoring multiple attributes
on the peptide level makes MAM a more informative
and streamlined approach than typical LC and MS
methodologies. A recent publication by Millán-Martín et
al. described a comprehensive MAM workflow for biologic
characterization and current good manufacturing (cGMP)
practices.7
This work aims to standardize the protocols
used for MAM to help with implementation and is the first
protocol to establish the MAM methodology, which has
not been available in the literature prior. The overview of
the workflow explains the three major parts of setting up a
MAM protocol:
1. Sample digest: A proteolytic digest of the protein to
18 TECHNOLOGYNETWORKS.COM
Lab of the Future
Credit: iStock
produce peptides.
2. High-resolution accurate MS (HRAM-MS) of the
peptide: Discovery phase (phase 1) to enable confident
identification of peaks to produce a peptide library
for the biologic. Monitoring phase (phase 2), where
full MS acquisition is carried out for the predefined
product quality attributes (PQAs) and critical quality
attributes (CQAs) based on the phase 1 peptide library.
3. Data processing and analysis: Data processing for
phase 1 looks to evaluate the sequence coverage and
assess the PQAs/CQAs present for the biologic. In
phase 2, during targeted monitoring, the predicted
PQAs and CQAs are monitored, and detection of new
peaks are screened/investigated for the presence of
either product or process-related impurities that may
be present during bioprocessing.
This MAM method relies on a bottom-up approach. Other
technologies using MAM seek to do native MS to allow for
PQA/CQA analysis on the intact protein level, which can
give a different level of information. Work by Bhattacharya
et al. explains the use of native-MS MAM to characterize
antibody titer, size, charge and glycoform heterogeneity in
cell culture supernatant.8
The workflow was achieved by
2D-LC-MS in which the first dimension coupled Protein
A in series with size exclusion chromatography, and the
second dimension was cation exchange chromatography.
The glycosylation was then characterized with Quadrupole
Time of Flight Mass Spectrometry (qTOF-MS). Academic
research has seen the quick adoption of the use of MAM
workflows, which will help speed up workflows in industry
applications once validated for use.
Forecasting multistep-ahead-profiles using
data-driven models
Process models and simulations are increasingly used to
better understand, optimize, and predict different aspects
of a bioprocess. More dense datasets are now available for
bioprocesses with new advancements in process analytical
technology (PAT) and artificial intelligence (AI). By
integrating AI-based data-driven models with an upstream
bioprocess, the outcome can be forecasted with better
prediction. Outputs, such as the cell viability, product
titer and metabolite concentrations, such as glucose, can
be predicted and better optimized for the process. These
optimized models can also be implemented with a realtime digital twin.
Recently, researchers at Sungkyunkwan University
published a practical guideline for selecting optimal
combinations of data-driven elements to predict Chinese
hamster ovary cell culture’s process profile.9
This was
achieved through a systematic framework for collectively
evaluating various AI algorithms, forecasting strategies
and model inputs. The utility of this framework was
then demonstrated on an array of cell culture datasets
under various conditions, selecting the most predictive
algorithm for said condition. The choice of model elements
that are considered will impact both computation load
and accuracy of the model, the researchers found. The
suitability of the model will depend on data availability,
the complexity of the modeled culture conditions and
model input variables. This framework should be used as a
rational and practical guide on what should be considered
to build the best forecasting data-driven model.
References:
1. Tihanyi B, Nyitray L. Drug Discov Today Technol. 2020;38:25-
34.
2. D’Amico CI, et al. J Am Soc Mass Spectrom. 2023;34(6):1117-
1124.
3. Tiwold EK, et al. J Pharm Sci. 2023;112(6):1485-1491.
4. Lambiase G, et al. J Chromatogr A. 2023;1691:463809.
5. Zhang L, et al. J Chromatogr A. 2019;1602:317-325.
6. Fan J, et al. Sep Purif Technol. 2023;317:123920.
7. Millán-Martín S, et al. Nat Protoc. 2023;18(4):1056-1089.
8. Bhattacharya S, et al. J Chromatogr A. 2023;1696:463983.
9. Park S, et al. Biotech Bioeng. 2023;120(9):2494-2508.
Considerations for HTS
Artificial Intelligence and High-Throughput Technology
AUTOMATION MINIATURIZATION DATA ANALYSIS
The growing influence of AI across manufacturing can also be seen in biomedical settings. Let’s
take the example of drug discovery.
There are several points during a pharmaceutical’s life cycle where AI can have a say.
Target
identification
The majority of targets for small-molecule drugs are proteins.
Open-source software AlphaFold2 can predict the structure with incredible
accuracy.
AlphaFold uses an input amino acid template sequence to build up a multiple sequence
alignment model that predicts molecular arrangement.
This initial step is combined with neural networks – data structures that mimic the connections
between cells in the human brain.
Click here to download the full infographic
Cubis® II Lab Balance –
The Future of Lab Weighing
Experience unparalleled connectivity and efficiency in
your lab with the Cubis® II balance.
Seamlessly connect to LIMS/ELN systems or Ingenix fleet
management, ensuring the highest level of accuracy and
data integrity for your weighing data. Fully compliant with
Ph.Eur. Chapter 2.1.7, USP Chapter 41, and 21 CFR Part 11,
the Cubis® II ensures all standards are met, no matter the
industry. Connect your lab to the future with the Cubis® II.
Click to Learn More
21 TECHNOLOGYNETWORKS.COM
Data Reporting: Connecting the
Islands of Automation
Bob McDowall, PhD
In this article, we explore ways analytical scientists can
improve their data reporting and sharing practices, leading to
improvements in data integrity and regulatory compliance.
Laboratory process automation in the pharmaceutical
and related industries has been a topic of discussion since
the 1980s. To help achieve this, there have been many
technical advances in applications such as instrument
data systems (e.g., chromatography data systems (CDS)),
electronic laboratory notebooks (ELN), laboratory
execution systems (LES) and laboratory information
management systems (LIMS). In addition, there has been
convergence between applications; LIMS applications
integrated with LES functionality mean only one
application must be implemented.
However, often the problem is not the functionality offered
by an informatics application but the way a project is
implemented in laboratories. In many cases, informatics
application problems include:
• Failure to design a fully electronic process for
automation.
• Not eliminating spreadsheets from a process.
• Incorrect application configuration by overinterpreting regulations.
• Not interfacing instruments requiring manual data
entry into the application.
• Partial instead of end-to-end process automation.
We will discuss some ways of implementing these informatics
applications to avoid running into these problems.
Although the focus in this article will be on good practice
(GXP)-regulated laboratories, the principles outlined
here are applicable for all. Even if GXP regulations do not
apply, the business benefits of effective laboratory process
digitalization will enhance business effectiveness, speed of
decision making and collaboration inside and outside of an
automated laboratory.
The aim of this article is to connect the islands of
automation that exist in an ocean of paper in most
laboratories to ensure an electronic environment.
Process, process, process
The starting point for an effective implementation of any
informatics application is to understand the workflow that
you are automating. Typically, this is a two-stage process:
1. Map and understand the current (as-is) process
This needs to be a logical mapping of the process
with a team of subject matter experts (SMEs) from
the laboratory. They need to document the activities
in the process, including those not in current SOPs,
and understand the data inputs and outputs and
the records created. Any bottlenecks in the process
are identified and the causes listed. Paper printouts
and data vulnerabilities that need to be resolved
in the second part of the mapping process can also
be documented. Lastly, improvement ideas for the
process should be listed. Process maps can be drawn
after the workshop for review later.
Lab of the Future
Credit: iStock
22 TECHNOLOGYNETWORKS.COM
Lab of the Future
2. Redesign the process to work electronically (to-be
process)
The first part of the second phase is to review the
“as-is” process maps from the first phase. Inevitably
there will be changes and this is normal. Once the
current process maps are agreed, the improvement
ideas can be used to challenge the as-is process. This
includes removing bottlenecks, eliminating paper and
interfacing analytical instruments to avoid manual
data transfer and the associated transcription error
checking. The “to-be” process should be used as a
blueprint for automating the process with the selected
informatics application.
Although redesigning a process takes time, the payoff is
immense as the process is simplified and operates faster
than currently performed. If an application automates an
as-is process, there is a high likelihood that it will require
more effort as the process will still be hybrid and less
efficient with zero return on investment for the parent
organization. Therefore, management must resource and
support the process redesign effort.
Process mistake one: Partial automation
During training courses that I have conducted, several
attendees have stated that the first phase of a LIMS project
is to automate sample management alone. This is a mistake,
in my view, as a complete process is not being automated
and there will be no business benefit generated. There will
be a lot of effort being put into sample management with
pretty barcode labels but nowhere to use them during
analysis.
Instead, ensure sample management is integrated into an
end-to-end process that generates reportable results. This
is not to say “automate the whole laboratory” but rather
to ensure that say, raw materials testing can be automated
in its entirety from receipt to release. Then additional
analytical processes such as in-process, finished product
and stability testing can be added incrementally.
This approach is vital for demonstrating to both users and
management that the system works and delivers benefits.
Process mistake two: Failure to interface
analytical instruments
If no analytical instruments are interfaced to an
informatics application, how will analytical data be input
to the software? Manually, obviously! However, it is better
to now consider the impact of the updated EU GMP
Annex 11, where proposals for validated software technical
controls are preferred to procedural controls operated by
users:
Clause 2 ... Configuration hardening and integrated
controls are expected to support and safeguard data
integrity; technical solutions and automation are
preferable instead of manual controls.1
The regulation also states that digitalization should be
considered:
Clause 3. An update of the document with regulatory
expectations to ‘digital transformation’ and similar
newer concepts will be considered.1
In addition to the business benefits of interfacing
now, there is the probability of regulatory pressure
to automate in the future. Therefore, when planning
laboratory automation, it is essential, from both business
and regulatory perspectives, that key instruments are
interfaced in the first phase of any project.
Process mistake three: Failure to eliminate
spreadsheets
Spreadsheets are ubiquitous in laboratories, easily
available, easy to use and a compliance nightmare.2,3,4
They are also a hybrid system with signed paper printouts
and an electronic file. The biggest problem is that as a
hybrid system you can’t have an electronic process. The
calculations performed should be incorporated into the
informatics application to prevent printouts and manual
input of the data.
The exception to this rule is where a spreadsheet can be
used within an application such as an ELN where the
application’s audit trail can monitor changes, but the
data used in the spreadsheet calculation must be loaded
automatically into the spreadsheet to avoid printing and
transcription error checking. However, it is critical to
check if the application audit trail can track individual
actions within the spreadsheet because if interim changes
between an open and save event cannot be tracked this
is a data integrity failure. You are just changing a hybrid
problem into an electronic problem.
Process mistake four: Failure to eliminate
blank forms
The FDA has required quality control (QC) laboratories to
control blank forms since 19935
and this has been iterated
in regulatory guidance on data integrity from MHRA,
FDA and PIC/S.6,7,8
If uncontrolled blank forms are used
it is impossible to determine how many times the work
has been performed before an “acceptable” result has
been obtained. If blank forms are used the administrative
controls required to ensure that data cannot be falsified
result in a high overhead for the QA department to issue
and reconcile these forms.
23 TECHNOLOGYNETWORKS.COM
Lab of the Future
Credit: Bob McDowall
Process mistake five: Failure to use electronic
signatures
Although a process may be automated, some laboratories
don’t take the final step of using electronic signatures by
the performer and reviewer of the analysis. This creates
a hybrid system but also leaves the electronic records
unlocked, at which point post-reporting changes could
be made. This is unacceptable and therefore the use of
electronic signatures is a natural outcome of an electronic
or digitalised process.
Turning principles into practice
That’s the theory for laboratory automation. To see how
this is put into practice, we interviewed Roger Shaw of
Digital Lab Consulting (DLC). His principles for enabling
laboratory connectivity, data sharing and regulatory
compliance are to:
• Ensure analytical instruments are connected to
instrument data systems or informatics applications.
• Connect informatics systems together and
communicate electronically between them.
• Ensure each informatics system supports applicable
regulatory requirements including data integrity.
From these principles, there are further requirements.
Instrument standardization is important as it simplifies
interfacing as well as a reduced validation approach that
can be taken after the first connection has been verified.
Unlike pharmaceutical manufacturing, where there are
well-established data standards and protocols, there is a
lack of data standardization and interface standards for
laboratory instruments. For some projects Roger’s team
have used instrument interfacing standards developed
by the SILA Consortium and data standards such as
ISA-88 when combining process development and
manufacturing data. For other projects, the data system
from the instrument supplier provides a simple interface
for connection.
Roger described a case study for a QC department in one
site of a multinational pharmaceutical company, shown in
Figure 1. The components of the project consisted of the
following:
• Gas and liquid chromatographs connected to a
networked CDS.
• pH meters and analytical balances connected to the
instrument supplier’s data system which is equivalent
to an LES in that workflows can be defined to link
instruments together for assays.
• LIMS connected to the CDS and instrument data
system.
Chromatography
Data System
HPLC
Analytical
Balance
pH
Meter
Laboratory
Information
Management
System
Instrument Data
System
Figure 1: Outline of a laboratory automation project.
24 TECHNOLOGYNETWORKS.COM
Lab of the Future
The workflows between the three informatics applications are:
• The LIMS is responsible for sample management and
reporting of results to production.
• The pH meter and balances are interfaced to the
instrument data system which acquires data from
standards and samples and transfers the results of this
work to the LIMS.
• The sample identities and weights, and the reference
standard purities are imported from the LIMS into the
CDS for each batch of analysis.
• Following chromatographic analysis and
interpretation, all post-run calculations are
incorporated into the CDS workflows and electronic
signatures are used by the performer and reviewer of
the batch.
• When a reviewer signs the CDS report, all records are
locked and can be viewed by authorized users but not
changed unless unlocked.
• The reportable result is transferred to the LIMS.
• All sample test results are collated in the LIMS and a
certificate of analysis (CoA) is generated electronically
and electronically signed.
Business benefits as well as regulatory compliance are
obtained with this approach.
Summary
This article explores ways to improve data reporting and
sharing practices coupled with improving data integrity
and ensuring regulatory compliance. To achieve these
goals, it is imperative that the current process is analyzed
to identify bottlenecks, use of spreadsheets and blank
forms and identify data vulnerabilities. The redesigned
process should eliminate as many these problems as much
as possible by working electronically with electronic
signatures.
To ensure success the applications selected to automate
the new process must have adequate technical controls to
ensure data integrity and regulatory compliance. Rather
than be standalone, each application should be interfaced
to transfer data and information electronically to enable
effective scientific collaboration.
References
1. European Medicines Agency. Concept Paper on
the Revision of Annex 11 of the Guidelines on Good
Manufacturing Practice for Medicinal Products –
Computerised Systems. 2022.
2. US Food and Drug Administration. FDA Warning Letter
Tismore Health and Wellness Pty Limited. 2019.
3. McDowall RD. LCGC Europe. 2020;33(9):468–476.
4. McDowall RD. Spectroscopy. 2020;35(9):27-31.
5. US Food and Drug Administration. Inspection of
Pharmaceutical Quality Control Laboratories. 1993.
6. Medicines and Healthcare products Regulatory Agency.
MHRA GXP Data Integrity Guidance and Definitions. 2018.
7. US Food and Drug Administration. FDA guidance for
industry data integrity and compliance with drug CGMP
questions and answers. 2018.
8. Pharmaceutical Inspection Convention/Pharmaceutical
Inspection Cooperation Scheme. PIC/S PI-041 Good
Practices for Data Management and Integrity in Regulated
GMP/GDP Environments Draft. 2021.
25 TECHNOLOGYNETWORKS.COM
The Future of Digital Health With
Professor Michael Snyder
Kate Robinson & Lucy Lawrence
Michael Snyder, Stanford W. Ascherman professor of
genetics, is a leader in the field of functional genomics and
proteomics.
Snyder has combined different state-of-the-art “omics”
technologies to perform the first longitudinal detailed
integrative personal omics profile (iPOP) and has used
this to assess disease risk and monitor disease states for
personalized medicine.
Technology Networks invited Snyder to an Ask Me
Anything session to answer your questions about the latest
technologies and innovations that are shaping the future of
digital health. These are just some of the questions that we
asked Snyder, click here to watch the full AMA.
Q: What sparked your interest in lab digitalization?
A: Well, I think the healthcare system is broken. We tend
to go to a physician only when we’re ill rather than try and
keep ourselves healthy.
There are many steps involved in healthcare. You have to
travel, usually at a very inconvenient time, to a doctor’s
office, which pretty much looks the same as it did 40 years
ago. When you’re there, they stick a needle in you and
draw blood, and from that blood, they’ll make very few
measurements. Then they’ll make decisions about your
health based on population averages, calculating a mean of
your measurements, and compare it with everyone else’s.
So, we think every one of those steps can be changed. And
I think one big part of it is pulling in big data.
Q: How can wearable technology bridge the gap
between lab research and clinical applications?
A:Right now, this is all research, but a small number of
wearable devices are approved by the US Food and Drug
Administration (FDA).
The reason we’re very keen on wearables is because they’re
measuring you 24/7. In a physician’s office, you get 15
minutes, then they make a measurement, and most people
are anxious, so the measurements aren’t always accurate.
Wearables run in the background and measure heart rate
and heart rate variability, which are important health
monitors, and because they can take your resting heart rate
first thing in the morning, they’re quite accurate.
So, if something is off, it’s likely that you’re either ill
mentally or physically.
When I had presymptomatic Lyme disease, my smartwatch
showed that my heart rate jumped up and my blood oxygen
dropped, and after blood tests I was given a diagnosis.
In a real-time detection study, we have also shown that
you can tell someone with COVID-19 is unwell. Our realtime alerting system can detect illness three days prior to
symptom onset, and it’s more sensitive than an antigen test
in some cases.
Lab of the Future
Credit: iStock
26 TECHNOLOGYNETWORKS.COM
Lab of the Future
Credit: iStock
If you’d like to sign up to a study, follow this link: https://
innovations.stanford.edu/wearables
Q: What do you see being the future of digital health
and lab tech, relating to diabetes?
A: Historically, glucose monitors have mainly been useful
for type one diabetics and insulin dependent type two
diabetics, but we started putting these monitors on people
without diabetes and those with prediabetes.
In doing this, we discovered that a lot of “normal” people’s
glucose spiked just as high as those with diabetes, which
could be an indication of those on their way to becoming
diabetic.
Glucose monitors are powerful, because what spikes an
individual’s glucose is very personalized, meaning some
people spike in response to bananas, others to potatoes,
etc. Nearly everybody spikes in response to rice and
cornflakes. These differences are partially due to the
microbiome, along with genetic and epigenetic factors.
Apps have been developed that utilize continuous glucose
monitors to make personalized recommendations on what
foods to eat, what to avoid, what times to eat and how to
exercise.
In Europe, glucose monitors can be bought over the
counter, but in the United States, you still need a physician
to order them. I think monitors will soon be accessed more
easily for everyone.
The ultimate goal is to get your glucose levels under control
early. While this may not prevent you from becoming
diabetic, it could at least delay things for a few years. That’s
what health monitoring is all about, trying to keep yourself
healthy as opposed to trying to fix yourself when you’re ill.
Q: Do you see virtual reality playing a role in digital
health?
A: We’ll certainly see a role in health in general. I think
that people would prefer not to go to a physician for most
things, but rather, want to know what’s going on right
there and then.
Virtual reality could allow people to choose a time
convenient for them to have a checkup, and they could go
to a physician in person for more serious things.
Using virtual health doctors mediated through virtual
reality could help people relate to things better.
Right now, we’re used to thinking in two dimensions and
that works fine, but as these new platforms come out,
we’ll likely get better about thinking of them in three
dimensions.
From a research lab standpoint, virtual reality would be
highly useful and more accurate for training than watching
a video.
Q: Will data commons, with consent of the
individual, be a good direction to go in?
A: Sharing data and aggregating data – with consent of the
individual – is for the good of all.
There are millions of people with health records who have
used medications. If you have a health condition, it would
be good to know about the other people with the same
condition, what drugs they have taken and the outcomes of
treatment. There’s a lot of data out there on that, but it’s not
shared.
Companies that pull in these kinds of data do exist.
They try and use the data for health recommendations,
something that’s been done a lot in an academic
environment, as well.
These days, most things are being done in a federated
fashion, meaning that each hospital doesn’t share their
data, but they give access to it. So, you can run a search on
the data, and they can tell you what their outcomes look
like, which is better than nothing.
It’s not as good as if everyone shared their data, but we’re
still working our way through this.
Professor Michael Snyder was speaking to Lucy Lawrence,
Senior Digital Content Producer for Technology Networks.
SOLUTIONS
DETECTION
FOR PFAS
PerkinElmer’s portfolio supports your
laboratory identifying and quantitating
PFAS in complex matrices, meeting
stringent regulations and reaching
the lowest detection limits.
The QSight Triple Quad LC/MS/MS
system consistently delivers the
throughput and productivity you need
in your analytical testing laboratory.
LEARN MORE
For more information visit
www.perkinelmer.com/category/pfas
28 TECHNOLOGYNETWORKS.COM
Lab Sustainability: A Holistic
Approach
Srividya Kailasam, PhD
Laboratories are complex spaces that cater to different
disciplines and are designed to carry out activities ranging
from wet chemical reactions and microbiological studies
to analysis using sophisticated instruments. They may
be operated as independent commercial establishments
(e.g., testing labs), or they could be part of educational
or research institutions, companies and hospitals. Given
this diversity, the need for heating and cooling, and the
presence of energy-intensive instruments, it is hardly
surprising that labs consume inordinate amounts of
resources and generate large volumes of waste. These
could be both solid and liquid and possibly infectious.
In the past few years, there has been an increasing
awareness of the environmental footprint of labs and a
growing trend toward “sustainable laboratories”.1
The
scientific community has started to focus on areas that
make labs sustainable. In a study published in 2022, My
Green Lab and Intercontinental Exchange Inc. (ICE),
identified that many pharma and biotech companies have
adopted zero carbon goals.
In this listicle, we explore how a holistic approach that
includes optimal lab design, environmentally friendly
equipment and instrumentation, and awareness about
the impact of labs, coupled with training and adoption of
sustainable practices, is required to make labs “green”.
Lab design
A laboratory’s design can have a vast impact on its
sustainability. Careful consideration when building, setting
up or renovating a laboratory can help to reduce wastage
and lead to improved energy efficiency.
A lab should be designed to increase the amount of natural
lighting and energy-efficient artificial lighting should be
used as a supplement to the daylight. Increasing natural
lighting is known to not only improve productivity,
but also save costs. A white or nearly white ceiling is
recommended for the proper distribution of natural
lighting. Dark bench tops and reagent shelves that make
the room seem darker must be avoided.
While a well-lit, air-conditioned lab is essential for
the proper running of sophisticated instruments, it is
important to turn off the lights and air conditioning when
the instruments are not in use. To ensure compliance,
team members can take turns switching off the lights and
equipment not in use. Regular light bulbs can be replaced
with LED bulbs. Temperature control can also be achieved
using chilled beams.
When designing a sustainable lab, factors such as its
size, layout and infrastructure flexibility must be borne
in mind to minimize energy consumption and adapt to
future technological changes. The use of modular and
reconfigurable furniture also helps in creating a sustainable
lab. Biosafety labs must ensure unidirectional flow of
materials and personnel and incorporate controls such as
Lab of the Future
Credit: iStock
29 TECHNOLOGYNETWORKS.COM
Lab of the Future
negative pressure zones and airtight doors and windows
to prevent highly infectious pathogens from escaping
into surrounding areas. Since all materials entering a
biosafety level 3 lab (BSL3) are considered biohazardous,
minimizing the materials (paper, media bottles, plastics,
etc.) brought into biosafety labs will reduce the necessity
to autoclave and/or dispose of these materials that could
otherwise be reused.
Several resources are available to help with planning the
creation of a sustainable lab. For example, a strategic
design framework, Green Lab, has been developed to
help create labs with minimal environmental impact
using recycled materials. These labs should be capable of
generating energy from renewable sources.2
Involving a wide range of stakeholders, applying the
highest possible sustainability standards and balancing lab
sustainability goals with the health and safety of personnel are
suggested as the three keys to designing a sustainable lab.3
Equipment
Labs depend on a huge variety of equipment, and careful
consideration during its purchase, use and disposal is
crucial in ensuring sustainability goals are met.
When upgrading or replacing existing instruments, certified
environmentally friendly options, such as those with
ENERGY STAR® and ACT (Accountability, Consistency
and Transparency) labels, should be purchased. The
Environmental Impact Factor (EIF) criteria form the basis
of creating the labels for life science products. These labels
provide scores for parameters such as renewable energy
used during manufacture, water consumed during use and
sustainability impact at the end-of-life stage. Lower overall
score indicates lesser overall environmental impact. In
addition, My Green Lab certification helps the labs get their
sustainability practices audited.
Choosing models that consume fewer resources, including
energy and solvents, helps to reduce the environmental
impact. For instance, smaller autoclaves with more efficient
vacuum generation systems consume less water and can be
used instead of a larger model.
Periodic maintenance of all lab equipment and instruments
must be carried out to ensure optimal performance
and energy efficiency. Keeping incubators, freezers,
refrigerators and cold rooms clean and frost-free will make
them more energy efficient and will increase the lifespan of
these products.
When possible, refurbished instruments can be used to
minimize the build-up of hazardous substances and other
products that cannot be recycled in the environment.
Manufacturers are increasingly investing in building a
circular economy for their instruments, offering incentives
for labs to return their old equipment, which can be
refurbished and sold on.
Operation
Laboratories can contain a wide range of energy-intensive
equipment that often requires continuous operation.
Subsequently, compared to office buildings, they typically
consume 5 to 10 times more energy per square foot.
Coupled with this, many processes depend on singleuse plastics and use vast amounts of water. Although it is
challenging for laboratories to try to match the resource
consumption and waste output of a space with such
different needs, several steps can be taken to improve their
operational efficiency.
Instruments such as chromatographs, mass spectrometers
and spectroscopes can be shut down when not in use.
Similarly, other equipment such as pH meters, balances,
centrifuges and water baths can also be turned off when
not in use. Equipment that doesn’t require continuous
use can be fitted with programmable outlet timers, which
could help to reduce equipment energy consumption by up
to 50%.
When in use, the sash of fume hoods should be kept in the
lowest possible position to improve exhaust efficiency and
thus save energy. Energy can also be saved by lowering
the airflow volume when the fume hoods are not in use.
When possible, ductless hoods that filter contaminated
air before recirculating it can be used. Operating ultralow temperature freezers at -70 °C instead of -80 °C saves
energy without being detrimental to the samples.
Labs should reduce, recycle and reuse plastic products
whenever possible. Consolidating orders for plastic
ware, glassware and chemicals for the different projects/
experiments running in the lab or for multiple labs, buying
smaller pack sizes or buying just pipette tips instead of
boxed tips can reduce the plastic waste in the lab.
Routine lab activities such as filtration, which is an integral
part of sample preparation, generates plastic waste such as
single-use syringes and filter cartridges. These and other
plastic wastes, such as pipette tips, tip boxes, syringes,
tubes and nitrile gloves, can be recycled instead of sending
them to landfills or incineration. When recycling, care
should be taken to disinfect biowastes and segregate these
along with other hazardous wastes from recyclable, nonhazardous plastic wastes. A lab case report documented
various approaches, such as reusing decontaminated plastic
tubes and using sustainable materials like reusable wooden
sticks for patch plating and metal loops for inoculation
that helped reduce and reuse single-use plastics in a
microbiology lab. After implementing these strategies, the
lab demonstrated a 43 kg reduction in plastic waste in 4
30 TECHNOLOGYNETWORKS.COM
Lab of the Future
weeks, in addition to reducing costs.4A report from MIT
demonstrates the feasibility of recycling clean lab plastics,
stating that the Environmental Health and Safety (EHS)
office collected nearly 280 pounds of plastic waste from
participating labs every week in 2022 for recycling.
To minimize water consumption, taps and other water
outlets can be fitted with spray nozzles or low-flow
aerators, and recirculating water baths used for cooling
reactions that can be carried out on a smaller scale. To
reduce water wastage, leakage from faucets, autoclaves,
water baths and any seepage from water pipes should be
attended to as soon as possible. Washing and autoclaving
should be done with minimal wastage of water. Scientists,
students and lab personnel should cooperate to ensure that
autoclaves are run at full capacity to reduce water as well as
energy consumption.
Less hazardous substitutes for chemicals and cleaning
supplies should be used whenever possible. Labs
and workspaces must be kept clean to prevent crosscontamination that leads to wastage. Simple actions like
proper labeling and storage of chemicals will enhance their
shelf-life and proper usage.
Experiments should be designed to derive maximum
information with minimum consumption of resources as
well as minimum waste generation. This will also reduce the
number of experiments and eliminate erroneous experiments.
Replacing paper-based lab notebooks, operating procedures
and test protocols with electronic records will help minimize
paper consumption. Computers and other office equipment
can be turned off when not in use.
Training
Educating stakeholders on the importance of sustainability
is a crucial requirement for achieving a green laboratory.
In addition to training the scientists on guidelines and
regulations, they must be sensitized towards the ecological
impact of indiscriminate usage of chemicals, glassware,
plastic ware and consumption of resources, especially
electricity and consequent generation of waste in the
laboratories.
The importance of education and capacity building in
“green chemistry” and “sustainable chemistry” for creating
greener and more sustainable processes has been described
by Zuin et al.5 Sustainability in Quality Improvement
(SusQI), developed by The Centre for Sustainable
Healthcare (CSH) adopts principles of Education for
Sustainable Development (ESD) such as “future thinking”
and “systems thinking” and “thinking creatively” in the
training programs for clinical laboratory professionals.6
Several resources are available online for learning and
disseminating information regarding lab sustainability.
LEAF or Laboratory Efficiency Assessment Framework,
an independent standard for good environmental practice
in labs designed by University College London, provides
training, a toolkit, resources and strategies for improving
the sustainability and efficiency of labs.
Behavior
As behavioral changes take time, training on lab
sustainability must be augmented with monitoring,
reminders and encouragement to implement practices
that will lead to smaller carbon footprints. Educational
institutions have started initiatives such as “Unplug”
and “Shut the Sash” competitions to encourage lab users
to adopt energy-saving practices. In the “Sustainable
Laboratories” report published by the Royal Society of
Chemistry, recognizing and rewarding initiatives and
actions that promote sustainability in the labs has been
listed as the first plan of action along with providing
resources, funds and advocating for change.
Leaders can make a difference by setting the right goals
and performance indicators on sustainability, encouraging
collaboration and, most importantly, walking the talk.
The “Green Labs Guide” published by the University
of Pennsylvania and sustainable research practices on
Princeton University’s EHS website provide a number
of strategies and checklists that can help lab managers to
make their labs sustainable.
Conclusion
Sustainability in a lab should become a way of life. It will
not only reduce the environmental impact, but also save
money in the longer term, offsetting the initial investments
for achieving sustainability. A “green lab” should be
the collective responsibility of all the team members. A
well designed and well maintained lab also contributes
to higher efficiency and productivity. Awareness and
taking simple steps can go a long way in improving lab
sustainability.
References
1. Durgan J, et al. Immunol Cell Biol. 2023;101(4):289-301.
2. Belibani R, et al. Progress in Sustainable Energy
Technologies Vol II. 2014:273-283.
3. Hersh E. Harvard School of Public Health. Three keys to
sustainable lab design to improve health and safety. 2019.
4. Alves J, et al. Access Microbiol. 2021;3:000173.
5. Zuin V, et al. Green Chem. 2021;23:1594-1608.
6. Scott S. Clin Chem Lab Med. 2023;61(4):638-641.
31 TECHNOLOGYNETWORKS.COM
Failing Faster, Succeeding
Sooner: Technologies That Are
Accelerating Drug Discovery
Anthony King
Most drug hopefuls fall flat. Approximately 90% of drug
candidates fail clinical development, costing hundreds of
millions to billions of dollars. That’s a gargantuan waste
of resources thrown into compounds that never reach
patients.
Drug discovery scientists at university labs and in big
pharma, along with patients and governments, want more
winners. One solution is to have drugs fail faster, fail earlier
and fail completely – while it sounds contradictory, this
could reduce costs and efforts invested into compounds
that ultimately never make it onto a patient’s prescription.
Researchers now have some helpful levers that they can
pull to fail better and boost success rates. One is laboratory
automation, where automated technologies take the place
of human hands in manual and time-consuming tasks,
making the research and development of possible drugs
quicker and easier. A second is artificial intelligence
approaches (AI), which divulge patterns in the myriad
sand grains of data available, such as linking nebulous data
patterns to the intervention of a potential drug compound,
that no human could realistically wrap their head around.
Both are being combined with an array of innovative
techniques in the world of drug R&D to open new
treatment avenues, which we’ll explore in this article.
In silico methods for early compound
evaluation
The early stages of drug discovery benefits most from AI
right now, says Professor Andreas Bender. “There’s lots
of machine learning in early-stage ligand discovery, but
the problem is always translation to the clinic,” meaning
taking the research from laboratory to patient bedside. An
analogy for ligand discovery is finding a key that fits and
turns a lock, usually a protein involved in a disease. How
the key and lock interact is pure physics and chemistry, and
this is something that algorithms and computers handle
well. There are treasure troves of public data that can be
reached into, such as ChEMBL, a curated database of
bioactive molecules with drug-like properties.
Bender taps in silico computer methods, essentially
experiments performed via a computer simulation, to
evaluate compounds early on. Such calculations can
instantly reveal that a compound’s chemical structure
will lead to its rapid clearance from the body, meaning
dosing will be a problem in patients. “Despite having huge
amounts of data, predictive models are always fallible. You
need experimental data to validate,” says Professor Miraz
Rahman, medicinal chemist at King’s College London, UK.
Lab of the Future
Credit: iStock
32 TECHNOLOGYNETWORKS.COM
Lab of the Future
Credit: iStock
But biology gets messier as you move towards humans,
which is why the big hurdle is often Phase II clinical trials,
when a drug is tested to see if it carries efficacy and can
make a difference to patients’ lives. Often the information
fed into an algorithm to try to improve on patient efficacy
comes from lab animals, but the algorithm usually does
not know about underlying conditions that impinge on
the results from the animals, such as the age, genetics
or sex of the organism. This situation is set to improve.
“Experimental datasets will increase over the next 5 to 10
years probably,” says Miraz. “This will enrich AI models
and will likely make the success rate significantly higher.”
That is not to say machine learning cannot help now.
AI crossed with folk medicine
Bender investigates natural compounds believed to have
medicinal qualities for their influence on the formation of
blood vessels, known as angiogenesis. He used machine
learning to identify such plant compounds from texts
online, where they are often described as molecules of
interest in folk medicines. Such botanical products might
prep the pace of blood vessel formation and benefit heart
attack patients, or they might choke off blood supply
to hungry tumors, helping cancer patients. “We tested
the compounds to see if they had an effect on blood
vessel formation,” recalls Bender, who collaborated with
colleagues in China. Zebrafish embryos – less than a week
old – were placed in trays with 96 wells before varying
doses of the compounds were added to each well. Zebrafish
embryos are transparent, and automation in the lab
allowed each embryo to be imaged using a microscope and
the data fed directly into an algorithm. This revealed which
compounds, and at what dose, influenced angiogenesis.
“You get good bang for your buck and quite a lot of
return from your time and effort,” Bender comments on
the pairing of AI and automation in this context. It’s an
approach that is well established, and advances at this stage
in drug discovery can help move compounds closer to use
in patients.
“What matters is getting better compounds
into the clinic. That’s where the real impact
comes from,” says Bender. “Meaning the right
compound and the right dose into the right
patients.”
When combined with automated and cell imaging and
other laboratory techniques, AI can also make great
inroads in identifying patient subgroups that might
benefit the most from a specific treatment. This has been
most notable for cancer patients, where researchers have
developed successful treatments of patients by pinpointing
drug targets on their tumors. This tailored approach
requires an in-depth understanding of their individual
disease, something machine learning can assist with. For
example, clinical data and computer tomographic images
have been combined to identify which patients with
colorectal cancer are likely to also develop metastatic liver
cancer, and how best to treat them. Beyond cancer, there
is also hope that machine learning can aid the discovery
of drugs for central nervous system diseases, as noted in a
recent review.
Conversely, in the 1990s, high-throughput screening
of compound libraries pinpointed many interesting
candidates, but after testing and moves towards patients,
the approach turned out to be largely disappointing in
terms of patient impact. Lack of disease understanding was
often to blame. “If you poke in the dark, you will reduce
your chances of success,” says Bender. Instead, AI works
Bufobufo gargarizans.
Cinobufotalin is the primary
component of Chan-Su, an
extract from the paratoid
glands of B. gargarizans.
Bender and colleagues found
cinobufotalin could inhibit
endoethelial tube formation
in vitro, but promoted
angiogenesis in zebrafish.
The findings suggest the
active ingredient has
unknown pharmacological
effects, which should be
explored in more detail.
33 TECHNOLOGYNETWORKS.COM
Lab of the Future
Credit: iStock
best if there is a working hypothesis on how to treat a
disease, as well as relevant data to feed into an algorithm;
then, in silico predictions can deliver testable hypothesis
which, followed by experiment, can then identify diseasemodifying compounds.
Beating drug resistance
One area of drug R&D that has particularly struggled is the
discovery of new classes of antibiotics. Microbes that are
resistant to existing drugs are deemed one of the top global
public health threats facing humanity by the World Health
Organization. With effective antimicrobials, medical
procedures such as cesarean sections, hip replacement,
cancer chemotherapy and organ transplantations will
become far riskier. “Globally, recent data indicates that
close to one million deaths per year are due to these types
of infections,” says Dr. Jose Bengoechea, a professor of
biomedical sciences at Queen’s University Belfast, UK.
Bengoechea has just embarked on a project funded by the
Medical Research Council in the UK that will use AI and
machine learning to find new ways of turning off microbial
defenses against antibiotics.
Previously, AI has been tapped to identify how microbes
gained resistance in healthcare settings such as hospitals.
Often this relies on sequencing genomes of the microbes.
But Bengoechea’s new project seeks previously unknown
protein targets on a troublesome bacterial species,
Klebsiella pneumoniae. This is a common bacteria found
in the environment and naturally inside and outside the
human body, but some strains can cause pneumonia,
wound infections and blood infections in hospital patients,
which are difficult to treat with standard antibiotics.
As part of his project, Bengoechea seeks new ways to block
mechanisms of resistance in bacteria by combining AI and
lab experiments. “This will allow us to make discoveries
faster than traditional approaches,” he says. He explains
that the problem with traditional approaches is that they
keep turning up the same drug targets: “We are getting
the same hits all the time.” AI brings the opportunity
to discover new ways to make Klebsiella susceptible to
existing antibiotics and our own defenses, which will be
cross-checked with existing drugs. “We will interrogate
libraries of drugs that already have received approval,”
says Bengoechea, “dramatically cutting the cost and
time it takes for a drug to be tested in patients.” Results
can then be checked in lab experiments. If AI with other
experiments riding in tandem succeeds in reducing the
defenses of K. pneumoniae, the lab will explore whether
the strategy can be deployed against other troublesome
organisms resistant to our antimicrobials.
Fewer animal tests, and mini-me cancers
Newer approaches that lean on AI and automation can
reduce the reliance on animal testing in drug discovery
and developments. In principle, animal tests have not
been accepted for cosmetics in Europe for some time,
while recently the US removed the rule whereby tests on
two animal species were needed before drugs could go to
human trials. “I see this as a step forward, because the bar
is so low,” says Bender. “Animal tests are not sufficiently
predictive.” Results from drug tests on rats and mice often
differ from each other, never mind from people, and it is an
oft used quip that we have drugs that cured many diseases
in mice, but failed where it matters – humans. Now, in a
move away from animals, many scientists tap previous
experiments for running in silico tests, coupled with new
lab experiments that don’t involve rats, mice or guinea pigs.
For example, an anti-cancer compound might be added to
cells in a dish or mini-organs (organoids) in 96-well or 384-
well plates. An automated imaging system tracks changes
to the mini-organ or monitors what happens inside of
the cells, to see if expected changes occur. Once upon a
time, potential cancer drugs were mostly tested on human
cancer cells by applying them to immortalized tumor cell
Nuclei, microfilaments and membrane particles
in HeLa cells, a prolific cell line that has been
central to advances in modern medicine.
34 TECHNOLOGYNETWORKS.COM
Lab of the Future
Credit: iStock
lines organized in single layers on a dish or suspended in
a flask. One of the most famous is HeLa, a prolific line of
cells that originated from cervical cancer cells taken from a
US woman – Henrietta Lacks – who died of cancer in 1951.
Biologists could keep these cells alive beyond a few days in
the lab, and experiments using them contributed greatly
to medical advances. However, a problem is that such
cells adapt to life in the lab. Worse, perhaps, layers of cells
are not the same as the 3D milieu of cells in most human
tumors, so that cell lines do not mirror real-life cancer –
and there is lots of variation within tumor types.
This is where organoids enter the story. Cancer organoids
are tiny three-dimensional (3D) tissues that can be
grown in a lab from patient tissue obtained during
surgery or biopsy. They are predicted to revolutionize our
understanding of patient-specific tumours. “If you want
to find a drug that works for a patient’s tumour, then you
make sure the organoids you are testing are as close as
possible to the patient,” says Dr Alice Soragni, who heads
a lab at the University of California, Los Angeles, that uses
organoids to better understand rare cancers. She seeds 96-
well plates with organoids taken directly from patients at
surgery. Handling error and variabilities in techniques are
kept to a minimum because of automation use and how the
organoids are placed into the wells as a ring.
Once the “mini tumors” are in place, Soragni hits the
button on an automated system that monitors them while
they are exposed to different doses of off-the-shelf drugs
over several days. In one set-up, she can use an imaging
technique called interferometry that allows her to weigh
and image the cells in real-time, to see how they are
responding to a treatment.
The Soragni lab is now also printing cells from patients
with rare sarcoma tumours, embedded in a support matrix.
“This is a high throughput platform powered by machinelearning based tools,” Soragni explains, “which allows us to
extract as much information as possible from the images.”
Her team seeks to leverage machine learning tools to link
tell-tale characteristics of tumor organoids, which would
not be picked up by eye, to their response to therapy.
Organoids – tied with automated approaches – are
making headway in other areas too. In a Chinese study,
researchers profiled the chromosomes of 84 pancreatic
cancer organoids to analyze their response to hundreds of
chemicals and five chemotherapies. Meanwhile, scientists
in Germany developed a human midbrain organoid
to screen 84 drugs, pesticides and other chemicals for
toxicity. Their fully automated set-up identified a flame
retardant and a pesticide as toxic to dopaminergic neurons
through analyzing microscope images. These neurons,
which release the neurotransmitter dopamine, are of
interest partly because their deterioration is the hallmark
of Parkinson’s disease.
Back in California, Soragni is also interested in a genetic
condition, neurofibromatosis type 1, which causes tumors
to grow under the skin of patients. The tumors do not
spread or become cancerous, but they can cause a patient
pain and distress. Surgery is one option, but Soragni has
obtained patient tumor samples to grow into organoids
in a quest for treatments. She is testing existing drugs
on these patient-derived organoids to try to help these
patients. “Automation makes this possible, because
manual screening would be technically challenging, time
consuming and labor-intensive, and could also suffer
from operator issues,” explains Soragni, referring to the
fact that individuals in a lab can vary in their techniques
and introduce variability. “Once you have automation,
everything becomes not only faster but also more robust
and easier,” she adds.
She is excited about the vistas that lab automation and
organoids open in terms of discovering new drugs, but
also in trying them out against tissue from an individual
patient. “We don’t even need that much information
about the tumor per se,” enthuses Soragni. “We can take
a tumor, shower it with different drugs and let the tumor
tell us what works best.” The ultimate beneficiary of AI and
automation should be patients, with newer therapeutics
brought to market that are more tailored to them as
individuals, boosting effectiveness and diminishing side
effects – the right drug for the right patient.
For Research Use Only. Not for use in diagnostic procedures. © 2023 Thermo Fisher Scientific Inc. All rights reserved.
All trademarks are the property of Thermo Fisher Scientific and its subsidiaries unless otherwise specified. AD001871-EN 0223M
Built for stability.
Built for performance.
Why compromise?
Thermo Scientific™ Chromeleon™ 7.3.2 Chromatography Data
System delivers vast improvements in performance, compliance,
and overall usability while increasing the resilience of network
operations. With greater processing power and additional
network structure, the entire CDS performance is amplified
while providing IT with all the tools for security, stability and
maintenance without compromising the needs of the lab.
It’s the best Chromeleon CDS yet.
Learn more at
thermofisher.com/chromeleon
36 TECHNOLOGYNETWORKS.COM
How To Future-Proof Your LIMS:
Handling Software Updates
Bob McDowall, PhD
The shortest part of a commercial LIMS life cycle is the
time between the selection, implementation (including
computer validation for regulated laboratories), user
training and roll-out for operational use. This was covered
in two earlier articles for Technology Networks covering
LIMS selection and implementation. More recently,
an article on How to Future-Proof Your Laboratory
Informatics Environment looked at a wider informatics
environment in a laboratory. In this article, we continue
the journey of how-to future-proof a LIMS by ensuring
that it is kept current with software updates. This is the
longest part of the system life cycle and the one that
must be managed proactively to get the most out of your
investment in the system and ensure that it is futureproofed. Here, the focus will be on updates to the LIMS
application itself rather than IT infrastructure and support
e.g., operating system patches or changes to the computing
platform.
The assumptions made in this article are:
• Laboratory processes have been mapped and
redesigned to eliminate paper and spreadsheet
calculations with use of electronic signatures
• Key instruments and laboratory computerized systems
are interfaced to the LIMS
• There is a current written Requirements
Specification(s) (User and Configuration) that
documents the LIMS processes and application
configuration
• A laboratory has access to a number of LIMS
environments e.g., DEV (development), TEST or
VAL (validation) and PROD (production) to enable
upgrades to be evaluated before they are implemented
LIMS installations
Before we can start discussing LIMS application software
releases and upgrades we have to consider that a LIMS has
been implemented in one of two ways:
• In-house or on-premises installation: The LIMS can
be installed on physical or virtual hardware located
either in the company data center or in an off-site
data hotel. The system, IT infrastructure and the data
stored on it is under the direct control of the company.
• Software as a Service (SaaS) Installation: The
software is leased from the LIMS company and is
operated on virtual infrastructure using a cloud
service provider. Put simply: your data on someone
else’s computer. There is no direct control by the
laboratory. The only control is via the performance
metrics defined in the agreement with the SaaS
provider.
These two different implementations lead to two vastly
different approaches to handling updates: one is voluntary,
the other mandatory. On-premises LIMS installations tend
to be static and SaaS LIMS are dynamic, as we shall see
now.
Lab of the Future
Credit: iStock
37 TECHNOLOGYNETWORKS.COM
Lab of the Future
Credit: iStock
Types of LIMS upgrades
All software needs to be maintained and updates can be of
two main types:
• Error or bug fixes: If not for users, all software
would be perfect! During operational use, software
defects, errors or bugs will be found and reported
to the LIMS supplier. These will be classified by the
supplier from fatal to cosmetic. Fatal and high impact
errors will be resolved relatively quickly, as a software
patch or hotfix will be released for users to install to
resolve the problem. Whilst implementing patches or
hotfixes needs to be controlled, there is no need for
major retesting or revalidation activities as the error
is typically localized; however, read the release notes
first to understand the impact of the fix.
• Increases in functionality: These upgrades are
in response to existing customer input for new or
improved functions or may also arise from potential
new customers who will only select the system if
additional specified functionality will be available.
These upgrades need to be handled more carefully
as changes in the way the system operates now
may impact current processes that could have been
extensively configured or customized. Again, read the
release notes and understand the changes and their
impact on the system.
On-premises installation and LIMS upgrades
An on-premise LIMS installation is the easiest to manage
and control software upgrades as the laboratory, quality
and IT are all involved with the process and it can take
place at a time to suit everybody. However, this can be an
Achilles heel as it can lead to deferring several updates as
the laboratory might be too busy or it is not seen as adding
value. In a GXP regulated laboratory the maxim is we have
spent so much time validating the system – DON’T touch
it! However, this is a complacent attitude as all commercial
software, including LIMS, has a defined lifetime. Many
software companies will support the current major release
and the previous one. Therefore, after a time software
maintenance is limited to known bugs only support.
I have known several laboratories that have left their
system static without any changes for a number of years
and either they have to support the system internally or
have a major upgrade and data migration project on their
hands. Neither option is an ideal situation.
It is much better to have frequent incremental upgrades
than take the Big Bang option to upgrades. Assessment
of the release notes and evaluation of the changes in a
DEV environment allow a laboratory to assess whether
any retesting or validation is actually needed. A LIMS
that has been configured (turning functions on or off and
parameterizing others) is easier potentially to upgrade
than a customized LIMS (using either a vendor supplied
language or a recognized software language). The latter is
more difficult especially if the customization rules of the
supplier have been ignored as regression testing should
be conducted to see if the upgrade has impact any of the
customized functions.
SaaS installation and LIMS upgrades
The situation with LIMS application upgrades is
completely different with a SaaS installation. Remember
this is your data on someone else’s computer and you
cannot control directly. Under the agreement you will be
required to take ALL upgrades to the system. You will
read in the agreement that there is no opt-out. Given an
Agile development process, this could mean a new upgrade
every 3–4 months. If you are a regulated laboratory, this is
a major a problem as you have to consider revalidating the
system with each new upgrade. Welcome to the validation
treadmill. Regardless of the environment a laboratory
works in, regular enforced upgrades must be managed
effectively and the overall process is shown in Figure 1.
Before signing the agreement, you did read and understand
what was involved with the acceptance and timing of
upgrades. You did, didn’t you?
Let’s assume that there are quarterly upgrades per year not
counting hot fixes, the following process should apply as
shown in Figure 1:
• At the start of the development cycle (shown in blue),
the new features for the release will be selected from a
backlog and developed (coding, testing and resolution
of errors) over the course of several two-week sprints.
• Towards the end of the second month, the new
features for the next version will be known and
information for the release notes prepared. These
notes must describe what has been changed in the new
release and these must be given to customers in good
time so that they can determine if they want to use the
new feature and, if so, understand the impact on their
processes. This and the test environment are shown in
yellow in Figure 1.
• A test environment must be available for customers to
assess the new release and decide if they will use any
of the new features offered. Note: you will get these
features regardless, whether you want them or not, as
this is a condition of the agreement signed earlier.
• The LIMS SaaS provider also needs to allow enough
time for a customer to evaluate the new features and
decide if they want to use them or not and to see that
38 TECHNOLOGYNETWORKS.COM
Lab of the Future
Credit: Bob McDowall
they work correctly. The period available for this will
be defined in the agreement between the two parties.
• A major problem with GXP regulated laboratories
is that normally the change control process is slow
plus the time required to generate and execute the
validation documentation requires a complete rethink
to stay within the agreement timelines. Validation
needs to get smarter and more flexible as this is
repeated every three months and you don’t get time off
for good behavior. Now do you understand the term
validation treadmill?
• After a defined period in the test environment, the
release will be pushed to the operational environment,
shown in green, whether you like it or not (remember
the agreement you signed with the supplier?)
I am not trying to put you off from a SaaS LIMS, I want
you to be prepared for reality.
Summary
Software upgrades are a way of life: use software – you
must manage upgrades proactively. You can take the
ostrich approach and ignore them all or just implement
bug fixes but if you find an unknown error when your
software is out of support this potentially could result in
a major project to upgrade. This can be avoided by small
incremental upgrades that require smaller and simpler
retesting or revalidation meaning that your LIMS is futureproofed.
Testing
Coding Release
Backlog
LIMS Supplier
Software
Development
SaaS LIMS
Test
Environment
SaaS LIMS
Production
Environment
Release Notes
Roll out of Version
To Customers
TIME CRITICAL
Figure 1: Process flow of a SaaS LIMS upgrade or hotfix release.
Sponsored by
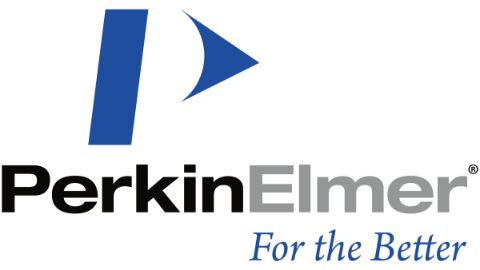
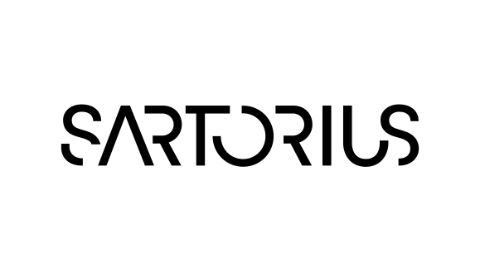
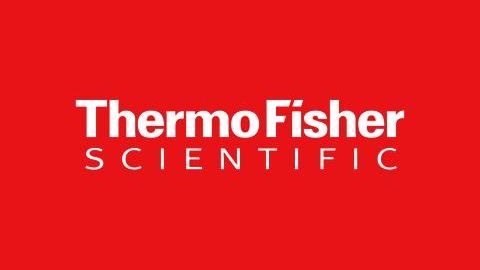
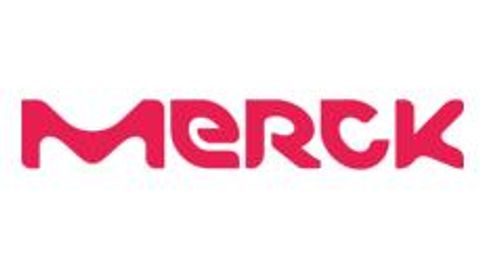
Download the eBook for FREE Now!
Information you provide will be shared with the sponsors for this content. Technology Networks or its sponsors may contact you to offer you content or products based on your interest in this topic. You may opt-out at any time.