Diagnostic Test Detects Ovarian Cancer With 93% Accuracy
Combining machine learning with blood metabolite data, researchers create a new diagnostic test for ovarian cancer.
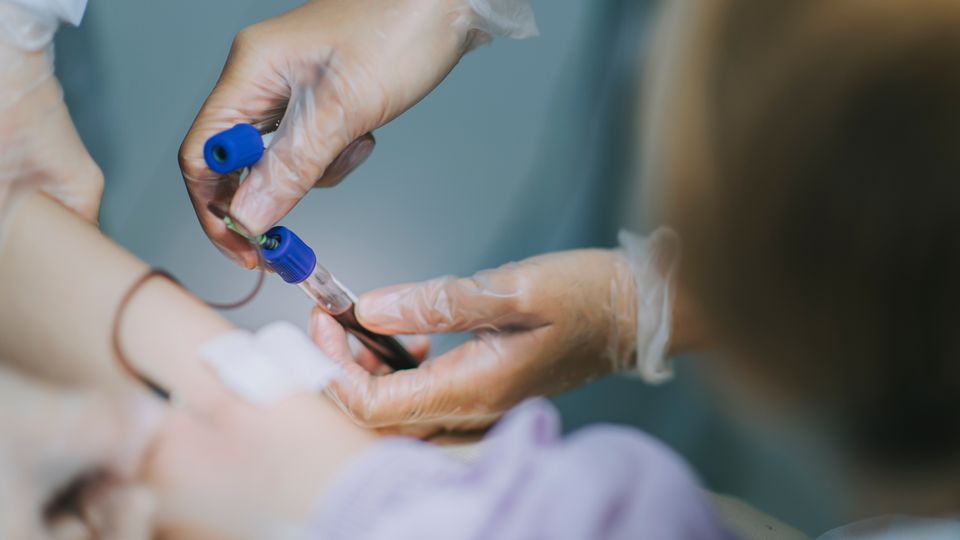
Complete the form below to unlock access to ALL audio articles.
Ovarian cancer – a “silent killer”
Ovarian cancer is often referred to as a “silent killer” due to the unfortunate fact that symptoms often arise once the disease has reached an advanced stage. By this point, effective treatment strategies can be limited. According to the Ovarian Cancer Research Alliance, the 5-year survival rate for patients diagnosed with stage I ovarian cancer is 89%; for stage IV, it’s 20%.
“Clearly, there is a tremendous need for an accurate early diagnostic test for this insidious disease,” Dr. John McDonald, professor emeritus in the school of biological sciences at the Georgia Tech Integrated Cancer Research Center (ICRC), said. McDonald is also the founding director of the ICRC.
Over the last three decades, there have been numerous efforts to create a highly accurate early-detection test for ovarian cancer, with limited success. That’s largely because cancer development is a highly heterogeneous process. While two patients might ultimately be diagnosed with the same type of cancer, their cells and tissues might have undergone very different molecular journeys to reach that point of diagnosis.
“Because of this high-level molecular heterogeneity among patients, the identification of a single universal diagnostic biomarker of ovarian cancer has not been possible,” McDonald said.
At the ICRC, McDonald and colleagues sought to identify and develop a machine learning-based classifier, which utilizes metabolic profiles of serum samples, to accurately identify people with ovarian cancer. The team’s research is published in Gynecologic Oncology.
Metabolic profiles in cancer
In metabolomics studies, mass spectrometry (MS) can help to identify what metabolites are present in a sample – such as blood – by detecting their mass and charge signatures.
What are metabolic profiles?
Metabolic profiles are a large set of biochemical markers and measurements that provide insight into an individual’s metabolic state. They might include information on the levels of circulating lipids, proteins, carbohydrates and other metabolites that can be harnessed to create a picture of an individual’s health.
MS only gets you so far, though. Identifying the exact chemical makeup of individual metabolites requires more extensive characterization, and only a small fraction of blood metabolites in the human body have been characterized. It’s not possible, therefore, to accurately pinpoint the molecular processes that underpin an individual’s metabolic profile – at least, not right now.
Even so, the presence of specific metabolites in the blood, as detected by MS, can be harnessed in the development of machine-learning based predictive models. “Because end-point changes on the metabolic level are known to be reflective of underlying changes operating collectively on multiple molecular levels, we chose metabolic profiles as the backbone of our analysis,” said Dongjo Ban, a graduate research assistant in the McDonald lab, and first author of the study.
“The set of human metabolites is a collective measure of the health of cells,” said co-author Professor Jeffrey Skolnick, “and by not arbitrary choosing any subset in advance, one lets the artificial intelligence figure out which are the key players for a given individual.”
Utilizing artificial intelligence to develop an early diagnostic test for ovarian cancer
To obtain the data to train their model, McDonald and colleagues collected serum samples from 431 ovarian cancer patients and 133 healthy women across 4 locations: Northside Hospital, Atlanta (10 early- and 142 late-stage cancer samples), Fox Chase Cancer Center Biosample Repository Facility, Philadelphia (51 early- and 68 late-stage cancer samples, 133 control samples), University of North Carolina Medical School, Chapel Hill (17 early-stage cancer samples) and Alberta Health Services, Alberta (23 early- and 120 late-stage cancer samples).
“To help ensure the quality of our metabolic data, individual normal and ovarian cancer patient samples were collected from four geographically divergent locations and analyzed using ultra-performance liquid chromatography coupled with tandem mass spectrometry (UPLC-MS/MS-positive and negative modes and each sample independently pre-processed through two columns), generating four distinct datasets,” the researchers described.
They then used recursive feature eliminiation (RFE) coupled with repeated cross-validation (CV) to identify the most reliable metabolites from the datasets.
What is recursive feature eliminaton and cross-validation in machine learning?
RFE is a method used in machine learning for feature selection, i.e., selecting a subset of the most important features from a dataset of features. In this study, “features” are the metabolites. In RFE, a model is trained on a dataset, where it ranks features based on specific criteria, and eliminates the least important features. This process is repeated several times.
CV is another technique that helps researchers evaluate the performance of machine learning models. By coupling RFE and CV, researchers can enhance the reliability of model evaluation and optimize feature selection.
McDonald and colleagues developed a consensus classifier – a final model – by aggregating the results of five independent machine learning algorithms. “The probabilities assigned to individuals by the consensus model were utilized to create a background distribution of probabilities that a given sample was cancer or normal,” the researchers explained.
Model distinguishes cancer from controls with 93% accuracy
The consensus classification model was able to distinguish cancer from control samples with 93% accuracy, according to the researchers.
“This personalized, probabilistic approach to cancer diagnostics is more clinically informative and accurate than traditional binary (yes/no) tests,” McDonald said. “It represents a promising new direction in the early detection of ovarian cancer, and perhaps other cancers as well.”
Want more breaking news?
Subscribe to Technology Networks’ daily newsletter, delivering breaking science news straight to your inbox every day.
Subscribe for FREEThe model requires further refinements and analyses. Its accuracy in predicting women with ovarian cancer was “slightly greater” than its accuracy in predicting women without the disease, the researchers explained in the paper. Currently, they do not know why, though they suggested that it could be due to the model potentially detecting disease in women prior to clinical symptoms and diagnosis. “Time course studies are currently being instituted to test this hypothesis,” they said.
Reference: Ban D, Housley SN, Matyunina LV, et al. A personalized probabilistic approach to ovarian cancer diagnostics. Gynecol Oncol. 2024;182:168-175. doi: 10.1016/j.ygyno.2023.12.030
This article is a rework of a press release issued by the Georgia Tech Integrated Cancer Research Center. Material has been edited for length and content.